Office of Pharmaceutical Manufacturing Assessment (OPMA)
Office of Pharmaceutical Quality
Abstract
Since the publication of the FDA’s PAT Guidance in September 2004, significant progress has been made in many aspects which boosted innovation in pharmaceutical development, manufacturing, and quality assurance. Most notably, it includes: (1) development and commercialization of novel technologies including measurement, analysis, and control technologies, which enabled real time process monitoring and data acquisition; (2) novel applications of PAT sensor technologies to numerous unit operations of various pharmaceutical dosages in development and manufacturing for better process understanding and process control strategies; (3) novel applications of PAT sensor technologies and chemometrics to facilitate Real Time Release Testing (RTRT) of pharmaceuticals as part of a manufacturing release strategy; (4) integration of PAT and data systems to facilitate systemwide active process control, and knowledge management, to maintain state of control of advanced manufacturing processes including continuous manufacturing, to improve and enhance manufacturing process robustness, quality improvement, and enterprise productivity improvement, etc. In this article, key achievements of those areas are summarized with emphasis on process monitoring and control strategy development. In terms of actual implementation of integration of PAT and data systems for pharmaceutical manufacturing and quality improvement, significant progress has been made in several vital areas, including but not limited to (1) Identification of critical product attributes (CQAs) and critical process parameters (CPPs) during pharmaceutical development as the first step of advanced process monitoring and control implementation; (2) Applying PAT for control strategy establishment across scale; (3) Applying PAT for continuous manufacturing control strategy establishment across scale; (4) Applying automatic control and data systems for integrated manufacturing system (IMS) and flexible manufacturing system (FMS) control strategy establishment, etc. In addition, some of the unprecedented opportunities for applying PAT for continuous improvement during development, scale-up, and post-approval change control of Immediate Release (IR) and Modified Release (MR) dosage forms has been discussed briefly. Furthermore, to tackle the overwhelming data challenges anticipated during control strategy establishment for advanced manufacturing implementation, such as the dynamic nature of data generation in terms of volume, velocity, and variety, apparently it is necessary to integrate PAT and data systems for effective manufacturing process control and product quality improvement. In the spirit of stimulating more discussions in this rapidly evolving area, with illustrations of two scenarios of integration of PAT and data system for manufacturing process control, challenges and opportunities along with certain considerations on quality, risk mitigation, and compliance have been discussed. Last but certainly not least, it is important to recognize that the knowledge gained from pharmaceutical development, realtime or frequent monitoring, and control strategy establishment could constitute the critical elements of modern pharmaceutical manufacturing control and release, enhance the science and risk-based regulatory assessment, and help the public to gain access to medicines with improved quality.
Disclaimer: The views and opinions expressed in this article are only of the author and should not be construed to represent FDA views or policies.
Introduction
Since the publication of the FDA’s PAT Guidance1 in September 2004, significant progress has been made in many aspects which boosted innovation in pharmaceutical development, manufacturing, and quality assurance. Dr. Janet Woodcock, the Center Director of the FDA Center for Drug Evaluation and Research, discussed what constitutes acceptable quality of pharmaceutical products:2 “Operationally, FDA considers high-quality drug products to be those that consistently and reliably deliver the clinical performance and other characteristics stated in the label, are not contaminated, and are available.” FDA CDER Office of Pharmaceutical Quality defined, (1) A quality product of any kind consistently meets the expectations of the user, and drugs are no different; (2) Patients expect safe and effective medicine with every dose they take; (3) Pharmaceutical quality is assuring every dose is safe and effective, free of contamination and defects; and (4) pharmaceutical quality is what gives patients confidence in their next dose of medicine. Therefore, from promoting and protecting the public health perspective, ultimately, any innovation in pharmaceutical development, manufacturing, and quality assurance should have the aforementioned merits. In this article, the current status of overall pharmaceutical manufacturing innovation where PAT is involved for manufacturing process control and quality improvement is summarized. One representative aspect of modern integrated pharmaceutical manufacturing process control, (i.e. integration of PAT and data systems to meet challenges of advanced manufacturing) is discussed from a technical and regulatory perspective.
Overview of Relevant Regulatory Guidance Documents on Modern Pharmaceutical Manufacturing and Quality Innovation
Pharmaceutical manufacturing has been dominated by batch processing for over a century. It has been recognized that significant opportunities exist for improving pharmaceutical development, manufacturing, and quality assurance through innovation in product and process development, process analysis, and process control. At the time FDA’s PAT Guidance was published in 2004, key high-level methodologies such as process understanding, process adjustment to accommodate variability, feedback and/or feedforward control had been advocated for better variability management and process robustness enhancement. Several principles and tools such as PAT tools, risk-based approaches, integrated system approach, and real time release were advocated. Subsequently, ICHQ8(R2)3 formulated the design space concept which can be established by application of, for example, formal experimental designs, process analytical technology (PAT), and/or prior knowledge. In 2011, FDA’s Process Validation Guidance4 highlighted general considerations, process design, process qualification, and continued process verification. In terms of establishing a strategy for process control, the FDA’s Process Validation Guidance recognized that process knowledge and understanding are dynamic, grow with increasing commercial manufacturing, and should be consulted on an ongoing basis for establishing the approach to processes control for each unit operation and the entire manufacturing process. Strategies for process control can be designated to reduce input variation, adjust for input variation during manufacturing (and so reduce its impact on the output), or combine both approaches. Interested readers are referred to the 2011 FDA Process Validation Guidance4 for the considerations on process design, process qualification, and continued process verification.
In 2019, the FDA published its draft guidance on continuous manufacturing.5 The FDA recognized the importance of process dynamics for defining batches of continuous manufacturing (CM) processes. Key considerations for control strategy include the following key elements: (a) Input Material Control; (b) Process Monitoring and Control; (c) Material Diversion; (d) Real Time Release Testing; (e) Specification; (f ) Equipment; (h) System Integration, Data Processing, and Management.
The FDA Process Validation Guidance4 is applicable to CM process.5 In addition, there are pharmaceutical quality system6 and stability7-8 considerations which can help to mitigate the overall risk at the system level and during product lifecycle. A 2017 FDA paper9 indicated that “the process analytical technology (PAT) initiative from the turn of the 21st century provides the foundation of continuous manufacturing while the QbD (Quality by Design. Note added by the author) effect of the past decade further fostered the development and adoption of continuous manufacturing for pharmaceuticals.”
Current Status of Overall Pharmaceutical Manufacturing Innovation Where PAT Implemented
The implementation strategies for pharmaceutical manufacturing and quality innovation where PAT is deployed has been advanced in various aspects. It can be categorized as follows:
- Development and commercialization of novel technologies including measurement, analysis, software, and control technologies, which enabled real time process monitoring and data acquisition.
- Novel applications of PAT sensor technologies to numerous unit operations of various pharmaceutical dosage forms for better process understanding and control strategies.
- Novel applications of PAT sensor technologies and chemometrics to facilitate Real Time Release Testing (RTRT) of pharmaceuticals as part of batch release strategy.
- Integration of PAT and data systems to facilitate system-wide active process control, knowledge management, maintain state of control of advanced manufacturing processes including continuous manufacturing, improve and enhance manufacturing process robustness, quality improvement, and enterprise productivity improvement.
A brief summary and discussion for each category is made below.
PAT technology advancement boosted real-time data acquisition and process monitoring
Novel technologies include real-time measurement, analysis, and software technologies. Differentiated from traditional off–line techniques, novel measurement and analysis technologies have been applied to measurement and control of critical process parameters (CPPs) and critical quality attributes (CQAs) in real-time, such as:
- Physical parameters including particle size via laser diffraction and/or FBRM, disintegration, particle morphology, etc. Chemical and/or physicochemical parameters including Identification and quantification via various spectroscopic and non-spectroscopic techniques10-12 (e.g., NIR, FTIR, THz, Raman, UV, thermocouples, manometric temperature measurement (MTM), etc.) and chemometrics
- Microbiological parameters including rapid microbiology screening in pharmaceutical workflows.13
Integration of novel PAT tools with pharmaceutical unit operations enabled better process understanding and advanced process control strategies
The focus of this type of implementation has been applying various novel PAT tools to pharmaceutical manufacturing to map the individual unit operation and/or entire manufacturing process for process and product understanding, design space, and control strategy establishment. The pharmaceutical community has witnessed innovation and creativity in applying various spectroscopic and non-spectroscopic techniques to pharmaceutical unit operations for real-time or frequent process monitoring and control.14-62 Table 1 highlights the CPPs and CQAs for several key unit operations which can be monitored and/or controlled via novel PAT tools.
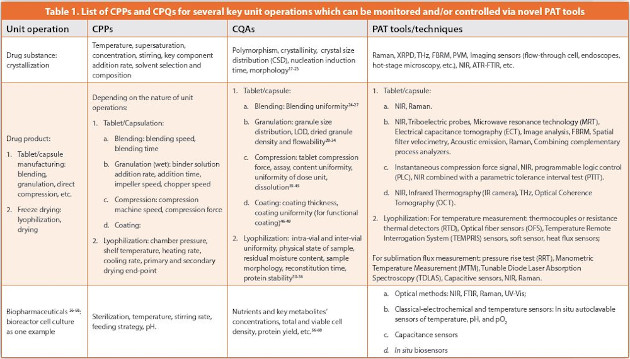
Compared to small molecule manufacturing process, bioprocess real-time PAT monitoring has certain unique technical challenges56-62 due to the enormous complexity of cellular systems and specific requirements of aseptic conditions, high number of analytes, drift, and often rather low physiological relevance, and strong interaction between recombinant gene expression and host cell metabolism. In addition, the typical run time of key bioprocessing unit operations such as bioreactor cell culture is much longer than its counterpart in small molecule drug product manufacturing. Therefore, the bioprocessing PAT probe has to be able to perform reliably and consistently over the prolonged manufacturing period, which poses challenges for probe design and performance qualification. In the meantime, establishing Multivariate Data Analysis (MVDA) models to reveal “hidden” information contained in the online data and thereby creating correlations to the multitude of highly specific biochemical off -line data has gained increasing popularity over the past decade. Exciting progress has been made in the abovementioned challenging areas.56-68 Previously, the feasibility62 of using a stepwise approach was demonstrated to integrate FTIR real time monitoring with chemometrics to establish a multivariate process control (MPC) modeling strategy for a monoclonal antibody (mAb) bioreactor cell culture process real time monitoring and prediction. All key metabolites and protein concentrations could be monitored and predicted in real time during the bioreactor cell culture process, which, for example, could help to deploy a rational feeding strategy, diagnose if any abnormal process behavior occurs (and if so, to what extent), and/or if the process is under state of control, etc. It demonstrated that modelling can not only enable the continuous prediction of otherwise discrete off -line generated data, but also enable critical process states being diagnosed and detected more easily. Figure 1 shows that the prediction profiles agree well with the experimental measured profiles for the key metabolites.
Implementation of Real-Time Release Testing (RTRT) as part of manufacturing release strategy
As discussed previously, the RTRT concept has been applied in regulatory approval of certain batch processes and continuous manufacturing processes. There are several discussions on RTRT available, including regulatory perspective69-70 and scientific case studies from small-molecule and biopharmaceuticals which demonstrated how RTRT can be facilitated by integrating manufacturing and multivariable control strategies to enhance and assure the quality of products.71-72 In principle, data streams from an integrated manufacturing process can be continuously used to make predictions and inform control systems. Juan L. Torres72 depicted a case study on how PAT and real-time multivariate analysis for trending, actions and disposition decisions can be integrated to establish drug substance advanced process controls.
Integrating PAT with data systems for pharmaceutical manufacturing
PAT is a highly integrated cross-disciplinary area. PAT should not go without data analysis and control algorithms, as both have been playing unique yet critical roles in process data analysis and control execution, including but not limited to: (1) basic multivariate analysis chemometrics (PCA, PLS, PCR, etc.) packages have been applied to establish multivariate models which linked raw materials’ properties, critical process parameters (CPPs), and critical quality attributes (CQAs)73-74; (2) advanced control algorithms (e.g., feedback forward/ feedback control, automated control strategy, proportional integral (PI) controller, etc.)75-79 have been applied for process automation, manufacturing robustness, and continuous manufacturing; and (3) more advanced algorithms such as pattern recognition and artificial neural network (ANN),80 deep learning and artificial intelligence (AI),81-85 etc., have been used to model complex patterns and problems including predictive maintenance for electrochemical sensors,81 medical imaging processing,82 materials degradation,83 etc. However, it should be noted that significant regulatory challenges76 for AI systems exist, if we consider that the intent and causation may fail to function because of the nature of the machine-learning algorithms on which modern AI are commonly built, and that it may not be possible to truly understand how a trained AI program is arriving at its decisions or predictions. The references cited here72-85 is just a short list and not meant to be exhaustive.
In terms of ultimate process control strategy development and implementation (i.e., advanced manufacturing), significant progress has been made in pharmaceutical and related industries. Some of the examples include: (1) advanced process control (APC),86-89 e.g., feedforward, decoupling, and inferential control, multivariable model predictive control (MPC); (2) intelligent control,90 e.g., a class of control technologies that use various artificial intelligence computing approaches (neural networks, fuzzy logic, machine learning, and genetic algorithms). In addition, statistical process control (SPC)91 as a method for monitoring, controlling, and improving a process through statistical analysis, has been widely used in the semiconductor industry and increasingly used in the pharmaceutical industry. Today, PAT serves as a critical element to pharmaceutical continuous processing, such as:
(1) to modulate or adjust the process to accommodate materials property variability and/or process perturbance, in-line and real-time process monitoring and product testing with feedback loops to the controller is essential. PAT tools which may be adopted for such usage include all real-time spectroscopic tools, real-time non-spectroscopic tools, intelligent control tools, and RTRT tools.
(2) to allow for online raw material release, eliminating or drastically reducing the wait time for chemical testing. PAT tools which may be adopted for such usage include rapid spectroscopic identification and quantification tools.
(3) to collate the sensor data streams for full electronic batch records and maintain correlation with processing parameters. PAT tools which may be adopted for such usage includes SPC, Multivariate Data Analysis (MVDA) techniques, SIMICA-P, etc.
Given all the above-mentioned progress in various critical aspects of PAT development and implementation, some critical yet legitimate questions for the community to consider have been: (1) how PAT can bring pharmaceutical processes from a 3sigma operation to a 6Sigma one? and (2) what steps may we take to further facilitate PAT implementation in pharmaceutical manufacturing in the context of robust control strategy? Obviously, those are open questions and challenges for the community to collectively address. The author made an attempt to review the current status and some of the critical challenges we are facing, in the spirit of stimulating more scientific and technical discussions in this evolving area.
Identification of critical product attributes (CQAs) and critical process parameters (CPPs) during pharmaceutical development as the first step of advanced process monitoring and control implementation
Development is the first stage in identifying critical product attributes and process parameters, which can serve as the foundation for establishing a robust control strategy92-96 via Quality-by-Design (QbD) principles and integrated risk assessment approach. It was shown that a systematic scientific based risk ranking and filtering approach allows a thorough understanding of quality attributes and an assignment of criticality for their impact on drug safety and efficacy.96
Applying PAT for control strategy establishment across scale
From the manufacturing process point of view, undoubtedly, the effect of scale should be taken into consideration for control strategy establishment for commercial manufacturing scale, regardless of the manufacturing process modes (e.g., batch, continuous, or hybrid process) when applicable. An example97 is available for using in-line PAT approaches to understand, control, and rapidly scale up the production of a hydrogenation reaction and isolation of a pharmaceutical intermediate. A calibration-free, qualitative, scale-independent approach using in situ mid-infrared (MIR) spectrometry was used to determine the end point of a hydrogenation reaction in real-time. Based on the consumption of an intermediate species, the reaction rate was calculated at any given time during the reaction. The algorithm, coupled with understanding of the process, allowed the end point to be correctly identified in triplicate during scale-up of the process from 0.2 to 20 L scale. Another reference98 described a concept of reducing upstream processing scale-up risks using QbD-enabling platforms and PAT tools for a biopharmaceutical bioreactor cell culture process.
Over the past 16 years, in general PAT tools have demonstrated advantages for enhancing process understanding and reducing process development and scale-up time. With increased process and product understanding, combined with process monitoring and control, the number of experiments performed during scale-up can be reduced, thus potentially decreasing drug substance usage in pharmaceutical development and most importantly development timelines. Some additional examples of applying PAT for process scaleup study can be found in public literature.98-100
Applying PAT and data system for continuous manufacturing control strategy establishment across scale
The FDA CM Guidance5 listed certain scenarios related to scale up, such as increase run time and/or mass flow rate, etc. When appropriate-sized equipment is used in the manufacturing process development, process scale-up with continuous systems can be as simple as just running the unit for longer periods of time. In such case, all of the design space data will be directly applicable to production operations with no need for extrapolations of the effects of larger equipment or different operating conditions.98 However, as typically real-time process monitoring and control is an essential component for CM process, when the choice of increasing run time is selected to meet marketing demands, data acquisition and storage could bring additional challenges. On the other hand, if larger equipment is necessary, the traditional scaleup effect should be addressed.
Control strategy establishment for integrated manufacturing system (IMS) and flexible manufacturing system (FMS)
Other advanced manufacturing technologies such as integrated manufacturing platforms and flexible manufacturing systems have gained increasing attention in the past decade. To enable integrated manufacturing platforms,101-102 it appears legitimate that we may use computers to control entire manufacturing processes in real time. Such integration allows individual processes to exchange information with other performance measuring systems to drive consistency and stability in the production of products.
Flexible manufacturing system (FMS),103 i.e., a manufacturing system that contains enough flexibility to allow the system to rapidly react to production changes, has been introduced in an attempt to avoid the penalties associated with relatively small production runs in a fixed plant. Two categories of flexibility are generally considered: machine flexibility (i.e., allowing the system to be changed to produce new product types (for example, products with different designs) and to change the order of operations executed on a part) and routing flexibility (i.e., the ability to use multiple machines to perform the same operation on a part as well as the system’s ability to absorb large-scale changes, such as in volume, capacity, or capability). Most FMS systems consist of three main systems: a material handling system for optimizing the flow of parts, a central control computer for controlling material movement, and the working machines (often automated CNC (computer numerical control) machines or robots). The FMS concept has been discussed as a corporate strategy to support manufacturing capability for a portfolio of products with varying manufacturing processes, manufacturing scales, and campaign lengths during the product manufacturing lifecycle (e.g., clinical, commercial launch, commercial supply) via option of scale-up or scale-out,104 including single-use disposable bag technologies105 for biopharmaceuticals and novel facility design concepts (i.e., Facilities of the Future) such as podular and modular designs.106
It is our understanding for those advanced manufacturing technology pharmaceutical applications, the process monitoring and control mechanism establishments via either engineering principles and system design, or other innovative technology tools are necessary to ensure that manufacturing remains the state of control during manufacturing, such that ultimately consistent high-quality product can be produced throughout the manufacturing process before release.
Opportunities for Applying PAT for Continuous Improvement During the Development, Scale-Up, and Post-Approval Change Control of IR and MR Dosage Forms
A PQRI (Product Quality Research Institute) white paper100 discussed best practices for the development, scale-up, and post-approval change control of immediate release (IR) and modified release (MR) forms in the Quality by Design paradigm. The authors of that 2014 whitepaper, i.e., a group of experts from the pharmaceutical industry, academia, and the FDA reached a consensus of the personal views that PAT is part of an overall strategy of continuous improvement that enables continuous learning through data collection and analysis over product life cycle, including:
PAT process controls, controls integration, and information management, including process controls and model-based systems, control integration and information management
- Life cycle management through proactive process enhancement/optimization
- Use of PAT in supporting post-approval changes to IR and MR products, including R&D requirements for a PAT Application In order to support post-approval changes, any reductions, replacements, or removal of PAT
- Improvement in the control and characterization of APIs
- Advancements in instruments and methods used in control and characterization of APIs via PAT.
In a comprehensive fashion, the paper highlighted certain high-level issues of PAT implementation during development, scale-up, and post-approval of IR and MR product life cycle.
Overwhelming Data Challenges Encountered During Control Strategy Development for Advanced Manufacturing Implementation
Leveraging development data and knowledge into the control strategy establishment may facilitate science and risk-based regulatory assessment of pharmaceutical manufacturing. In this regard, substantial advancement on data system and cloud computing in the past few years has made it possible that a centralized advanced manufacturing management system can be integrated with common communication interfaces (e.g., OPC technology) and well-designed IT platforms to establish a highly automatic manufacturing process control scheme, which essentially can increase manufacturing efficiency, enhance performance consistency, and ensure better product quality.
Due to multiple interrelated aspects such as data acquisition, data storage and processing, modeling and control execution in real-time involved in the advanced manufacturing system, the process monitoring and control in manufacturing setting could be technically challenging. In addition, moving the real-time monitoring and control from individual unit operation to the entire manufacturing line, and further to a plant- and corporate-wide implementation, will generate a high dimensionality time-series data matrix, which poses data handling challenges due to unprecedent demanding features on three V’s: Volume (amount of data), Velocity (speed of data), and Variety (range of data types and sources).107
When PAT tools are applied to various unit operations, entire manufacturing lines, and a plant in an integrated fashion, a short list of the realistic technical challenges to be addressed may include: (1) data storage for all data sources; (2) communication between each PAT measurement and/or monitoring tool; (3) data format associated with each PAT instrument or measurement tool; (4) computation capacity and cost of computation for the purposes of model establishment, validation, process monitoring and control on an ongoing basis; (5) data traceability for fault diagnosis and root-cause analysis when a problem occurs. In addition, when machine learning (ML) algorithms or neural networks (NN) used for pattern recognitions from high dimensionality complicated multivariate time series data generated from real time monitoring and control in the advanced manufacturing program, the “large p, small n” problem108-109 may occur. Here p stands for the number of features (predictors), n stands for the number of observations (sample size). The “large p, small n” problem leads to insufficient degrees of freedom to estimate the full model, which could occur for biopharmaceutical applications. In contrast, for the “small p, large n” situation, if there are high correlations between predictors, the prediction performance is dominated by ridge regression.110 In both cases, statistical expertise on data handling and computation is much needed. In a recent publication,111 it was demonstrated that a ‘large N’ NIR-based PAT method can increase reliability of quality assessments in solid dosage manufacturing.
Typically, some sort of data pre-treatment steps such as data alignment and data cleaning are necessary to get the dataset ready for in-depth data analysis and modeling to achieve the final goal of process control. A recent paper112 reviewed current data science applications at various stages of the bioprocess life cycle, including their scopes and data sources.
Rapid technology advancements in PAT instrumentation, data science tools, control system, data system, and advantages of PAT tools in pharmaceutical development and manufacturing have been demonstrated over the past decade. Based on the information disclosed in the public domain,113-115 it is evident that quite a lot of pharmaceutical institutes and companies have been investing in integrating PAT and data systems for bringing all data together and providing real-time predictions of the product and process quality. Successful examples of integration of PAT and data systems can be easily identified from other industrial sectors such as petrochemical industry, automobile industry, and semiconductor industry, etc.
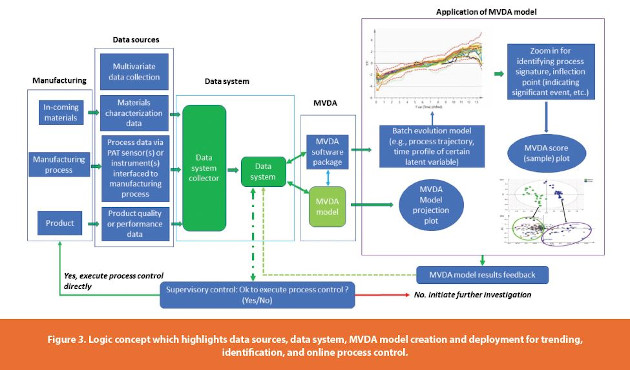
Based on the above-mentioned analysis, it seems reasonable to anticipate that integrating PAT, data pretreatment methods, and Multivariate Discriminant Analysis (MVDA) algorithms with data system or an information system provide an effective solution to the data challenge associated with advanced pharmaceutical manufacturing implementation.
PAT and Data System Integration for Process Control: Hypothetical Conceptual Integration Scenarios and Future Challenges
Two scenarios of integrated PAT and data system for manufacturing process control
Figure 2 describes a hypothetical conceptual scenario which illustrates integration of PAT and Data System for manufacturing process control.
To keep it simple, it only focuses on the integration of data flow and data system use. As such, interfaces among the manufacturing line, PAT sensor(s) or PAT instruments, computer, data system collector, Distributed Control System (DCS)/Programmable Logic Controller (PLC), data system central database (platform), and data system customers (e.g., process engineer, QC personnel, production personnel) were highlighted. In addition, essential functions such as remote monitoring, multivariate predictive monitoring, root cause analysis, automatic corrective recommendation, and real-time process control can be built-in to help to execute the control actions.
One of the advantages for such integration of PAT with data system lies in its capability to streamline the data acquisition, analysis, and modeling for ultimately achieving manufacturing process control and quality improvement. Compared to a standalone PAT instrument without a data system, it may free a MVDA (Multivariate Data Analysis) modeler from manual data exporting, tedious and error-prone data manipulation, and allow the MVDA modeler to synchronize time series data from different data sources for model building, storing, and deploying for real-time process monitoring and control, and quality improvement. Those technical features would allow pharmaceutical process engineers and scientists to take advantage of technology advancement and to use their core expertise to focus on critical aspects of manufacturing control strategy development and implementation.
Figure 3 describes another hypothetical conceptual scenario of integrated PAT and Data System for manufacturing process control. It shows several building blocks: (1) Manufacturing materials block; (2) Data sources block; (3) Data system block; (4) MVDA model block; (5) Application of MVDA model block; (6) Feedback and supervisory control block.
For illustration purpose, it included various capabilities of MVDA model after creation. After data acquisition, MVDA model will be calibrated, validated, and saved at the data system for deployment to perform functions such as trending, pattern recognition, online process monitoring and control. The MVDA modeling results, when fed back to the data system, can help the supervisor to determine if a proposed process control should be executed or an investigation should be initiated. The integration, if implemented successfully, could enable real-time prediction, quality improvement, and continuous verification.
Some considerations for integration of PAT and data system: Quality, risk mitigation and compliance
Based on the previous discussion, it is clear that integration of PAT and a data system is an evolving yet technically challenging area. When the integration of PAT and a data system is established for manufacturing process control and improved quality, the general scientific and regulatory assessment of manufacturing process, modeling, and facility will be conducted via a science and risk-based approach as per applicable relevant regulatory guidance documents, guidelines, standards and best practices, to ensure an overall control strategy commensurate with the associated risk. Some of the general considerations for integration of PAT and data system in the aspects of quality, risk mitigation, and compliance are provided below.
The initial list of CPPs and CQAs should be documented and justified by prior knowledge and initial risk assessment for each unit operation of the entire manufacturing process. If a broad Quality-by-Design (QbD) approach was adopted for pharmaceutical development and manufacturing control strategy establishment, it is important to keep all relevant data on manufacturing (i.e., in-coming materials, manufacturing process, and product release and stability available) in the data source to facilitate the establishment of linkage between raw material, process, and product (design space) as highlighted in Q8(R2).3 With pharmaceutical development, pilot-scale study, and other risk mitigation strategy applied, it is possible to narrow down the initial list of CPPs and CQAs further, such that it becomes practical to implement real-time or frequent PAT process monitoring and control, and quality improvement, given the current technologies available. It is understandable that when and how to implement real-time or frequent PAT process monitoring and control for pharmaceutical manufacturing control and quality improvement is up to the individual pharmaceutical company. When PAT and the data system is integrated for manufacturing process control and quality improvement, from a regulatory risk management perspective, it is essential to keep data traceability, especially for those data used for control strategy establishment and final release, as per relevant 21 CFR Part 11, Parts 210 & 211 cGMP requirements, Part 600, Part 820, and FDA/ICH Q7.116 In such case, a MVDA model or other type of control model are anticipated to be established as high impact model, as those models will be part of control strategy which directly impacts batch release and stability. Therefore, for development of such advanced integrated pharmaceutical manufacturing control systems, at design level, it will be necessary for the industry to consider several important features such as technical feasibility, scientific validity, control robustness, and fitness for intended use, etc.
Subscribe to our e-Newsletters
Stay up to date with the latest news, articles, and events. Plus, get special offers
from American Pharmaceutical Review – all delivered right to your inbox! Sign up now!
At the modeling level, it will be important for the industry to consider specific technical details such as rationale, protocol, and supporting data for model development, validation, maintenance, update, and change control (e.g., data traceability, data-pretreatment algorithms used, and version control), in order to ensure that executed control has been validated scientifically. For additional technical details regarding PAT-oriented modeling, referred to FDA draft NIR guidance117 for basic considerations of NIR model development, validation, and update as appropriate. For general PAT techniques involved, refer to ASTM E55.01 Process understanding and PAT system management, implementation and practice118 as appropriate. Refer to the most recent update of ASTM E2891-13119 for linkage between PAT model and manufacturing; Refer to the applicable user manual of the data system integrated.
To enhance the system integration performance, it is suggested the industry considers the following factors: (1) Establishing a system performance evaluation matrix ; (2) Conducting system sensitivity and robustness analysis for both internal and external disturbance to CPPs and CQAs which would have global impacts; (3) Establishing a user-friendly interface for visualization, data analysis, reporting, etc.; (4) Build-in data storage capacity, data security, and data backup function, etc.
Considering it is a conceptual open challenge, it is hoped that this discussion could stimulate more discussions from the community, such that collaborative effort can be in place to foster pharmaceutical manufacturing innovation and advanced manufacturing to meet unprecedented challenges for the ultimate purpose to promote and protect public health under a rapidly changing global environment.
Concluding Remarks
Significant progress has been made in many aspects which boosted innovation in pharmaceutical development, manufacturing, and quality assurance since the publication of the FDA PAT Guidance in 2004. In this article, key achievements of four critical areas during PAT implementation are summarized, with emphasis on process monitoring and control strategy development. In terms of actual implementation of integration of PAT and data systems for pharmaceutical manufacturing and quality improvement, significant progress has been made in several vital areas, including but are not limited to (1) Identification of critical product attributes (CQAs) and critical process parameters (CPPs) during pharmaceutical development as a first step of advanced process monitoring and control implementation; (2) Applying PAT for control strategy establishment across scale; (3) Applying PAT for continuous manufacturing control strategy establishment across scale; (4) Applying automatic control and data systems for integrated manufacturing system and flexible manufacturing system (FMS) control strategy establishment, etc. In addition, some of the unprecedented opportunities for applying PAT for continuous improvement during development, scale-up, and post-approval change control of Immediate Release (IR) and Modified Release (MR) dosage forms has been discussed briefly. Furthermore, to tackle the overwhelming data challenges anticipated during control strategy establishment for advanced manufacturing implementation, such as the dynamic nature of data generation in terms of volume, velocity, and variety, apparently it is necessary to integrate PAT and data systems for effective manufacturing process control and product quality improvement. In the spirit of stimulating more discussions in this rapidly evolving area, with illustrations of two scenarios of integration of PAT and data system for manufacturing process control, challenges and opportunities along with certain considerations on quality, risk mitigation, and compliance have been discussed. Last but certainly not least, it is important to recognize that the knowledge gained from pharmaceutical development, real-time or frequent monitoring, and control strategy establishment could constitute the critical elements of modern pharmaceutical manufacturing control and release, enhance the science and risk-based regulatory assessment, and help the public to gain access to medicines with improved quality.
Acknowledgement
I sincerely acknowledge very insightful comments from colleagues, especially Dr. Stelios Tsinontides and Commander Mahesh Ramanadham, to improve the article. The technical feedback on data system and presentation of Figures 2-3 from Tina Huang at OSIsoft is greatly appreciated. Moreover, I thank the FDA CDER Regulatory Science and Review (RSR) program for funding support on regulatory utilities of PAT in pharmaceutical development and manufacturing, the FDA CDER OPQ Office of Testing and Research (OTR) and the FDA CDER OPQ Immediate Office for providing opportunities to conduct laboratory-based PAT researches, and countless colleagues and friends in the pharmaceutical community for their support during my professional career development.
References
- FDA Guidance for Industry. PAT—A Framework for Innovative Pharmaceutical Development, Manufacturing, and Quality Assurance. September 2004. (accessed on 05/05/2020)
- Janet Woodcock. The Concept of Pharmaceutical Quality. American Pharmaceutical Review. Nov Dec 2004, 1-3.
- FDA Guidance for Industry. Q8(R2) Pharmaceutical Development. November 2009. (assessed on 05/05/2020)
- FDA Guidance for Industry. Process Validation: General Principles and Practices. January 2011. (accessed on 05/05/2019)
- FDA Guidance for Industry (Draft). Quality Considerations for Continuous Manufacturing. February 2019. (accessed on 05/05/2020)
- FDA Guidance for Industry: Q10 Pharmaceutical Quality System. (accessed on 05/04/2020)
- FDA Guidance for Industry: Q1A(R2) Stability Testing of New Drug Substances and Products. (Nov 2003). (accessed on 05/04/2020)
- FDA Guidance for Industry: ANDAs: Stability Testing of Drug Substances and Products, Questions and Answers. (May 2014). (accessed on 05/04/2020)
- L. X. Yu, M. Kopcha. The future of pharmaceutical quality and the path to get there. International Journal of Pharmaceutics, 528(2017) 354-359.
- L. Rolinger, M. Rüdt, J. Hubbuch. A critical review of recent trends, and a future perspective of optical spectroscopy as PAT in biopharmaceutical downstream processing. Analytical and Bioanalytical Chemistry (2020) 412:2047–2064.
- P. Bawuah, et al. Terahertz-Based Porosity Measurement of Pharmaceutical Tablets: a Tutorial. Journal of Infrared, Millimeter, and Terahertz Waves (2020) 41:450–469.
- D. Fissore, T. McCoy. Editorial: Freeze-Drying and Process Analytical Technology for Pharmaceuticals. Frontiers in Chemistry. Dec 2018, 6: 1-2 (Article 622).
- C. Surrette, et al. Rapid Microbiology Screening in Pharmaceutical Workflows. SLAS Technology 2018 August;23(4):387-394.
- H. Wu, et al. Monitoring, Understanding and Assessing Pharmaceutical Process and Product Quality. European Pharmaceutical Review, Dec 2015 (6), 50-56.
- T. De Beer, A. Burggraeve, M. Fonteyne, L. Saerens, J.P. Remon, C. Vervaet. Near infrared and Raman spectroscopy for the in-process monitoring of pharmaceutical production processes. International Journal of Pharmaceutics, 417(1-2), 32-47 1-13 (2011)
- S. M. Patel, and M. Pikal. Process Analytical Technologies (PAT) in freeze-drying of parenteral products. Pharmaceutical Development and Technology, 2009; 14(6): 567–587.
- Z. Nagy, M. Fujiwara, & R. Braatz (2019). Monitoring and Advanced Control of Crystallization Processes. In A. Myerson, D. Erdemir, & A. Lee (Eds.), Handbook of Industrial Crystallization (pp. 313-345). Cambridge: Cambridge University Press. doi:10.1017/9781139026949.011
- D. B. Patience and J. B. Rawlings. Particle-Shape Monitoring and Control in Crystallization Processes. AIChE Journal, 2001, 47(9): 2125-2130.
- H. Huang, P. Zavalij, L. Schultheis. Pharma Online XRD Analyzer. The National Institute for Innovation in Manufacturing Biopharmaceuticals (NIIMBL) Workshop, April 23, 2018, San Francisco, CA.
- Z. K. Nagy, G. Fevottec, H. Kramer, L. L. Simon. Recent advances in the monitoring, modelling and control of crystallization systems. Chemical engineering research and design, 2013, 91: 1903–1922.
- M. H. Bharati and J. F. MacGregor. Multivariate Image Analysis for Real-Time Process Monitoring and Control. Ind. Eng. Chem. Res. 1998, 37, 4715-4724.
- H. Wu. Continuous and discontinuous anti-solvent crystallization: an integrated real-time process monitoring and modeling approach. 2015 AIChE Annual Meeting, Salt Lake City, UT. Nov.10, 2015.
- Z.K. Nagy and R.D. Braztz. Advances and New Directions in Crystallization Control. Annual Review of Chemical and Biomolecular Engineering, 2012, 3:55-75.
- H. Wu and M. Khan. Quality-by-Design (QbD): An Integrated Approach for Evaluation of Powder Blending Process Kinetics and Determination of Powder Blending End-Point. Journal of Pharmaceutical Sciences. 98(8):2784-2798, 2009.
- A. S. El-Hagrasy, F. D’Amico, J. K. Drennen 3rd. A Process Analytical Technology approach to near-infrared process control of pharmaceutical powder blending. Part I: D-optimal design for characterization of powder mixing and preliminary spectral data evaluation. Journal of Pharmaceutical Science. 2006, 95(2): 392-406.
- H. Wu, M. White, M.A. Khan. An Integrated Process Analytical Technology (PAT) Approach for Process Dynamics-related Measurement Error and Process Design Space Development for a Pharmaceutical Powder Blending Bed. Organic Process Research & Development. 19(1) :215-226, 2015
- H. W. Ward, D. O. Blackwood, M. Polizzi, H. Clarke. Monitoring blend potency in a tablet press feed frame using near infrared spectroscopy. Journal of Pharmaceutical and Biomedical Analysis. 80:18-23, 2013.
- A. Kumar, K. V. Gernaey, T. De Beer, I. Nopens. Model-based analysis of high shear wet granulation from batch to continuous processes in pharmaceutical production – A critical review. European Journal of Pharmaceutics and Biopharmaceutics. 85:814-832, 2013.
- A. Burggraeve, T. Monteyne, C. Vervaet, J. P. Remon, T. De Beer. Process analytical tools for monitoring, understanding, and control of pharmaceutical fluidized bed granulation: A review. European Journal of Pharmaceutics and Biopharmaceutics. 83:2-15, 2013.
- E.M. Hansuld, L. Briens. A review of monitoring methods for pharmaceutical wet granulation. International Journal of Pharmaceutics. 472: 192-201, 2014.
- J. Clayton. Real-Time In-Line Monitoring of High Shear Wet Granulation. American Pharmaceutical Review. Dec 22, 2017.
- A.S. Narang, et al. Process Analytical Technology for High Shear Wet Granulation: Wet Mass Consistency Reported by In-Line Drag Flow Force Sensor Is Consistent with Powder Rheology Measured by At-Line FT4 Powder Rheometer®. Journal of Pharmaceutical Sciences. 105:182-187, 2016.
- J. P. Reddy, J. W. Jones, P. S. Wray, A. B. Dennis, J. Brown, P. Timmins. Monitoring of multiple solvent induced form changes during high shear wet granulation and drying processes using online Raman spectroscopy. International Journal of Pharmaceutics. 541: 253-260, 2018.
- V. Pauli, F. Elbaz, P. Kleinebudde, M. Krumme. Orthogonal Redundant Monitoring of a New Continuous Fluid-Bed Dryer for Pharmaceutical Processing by Means of Mass and Energy Balance Calculations and Spectroscopic Techniques. Journal of Pharmaceutical Sciences. 108: 2041-2055, 2019.
- L. Manley, J. Hilden, P. Valero, T. Kramer. Tablet Compression Force as a Process Analytical Technology (PAT): 100% Inspection and Control of Tablet Weight Uniformity. Journal of Pharmaceutical Sciences. 108:485-493, 2019.
- R. Singh, J. Zhang, M. Ierapetritou, and R. Ramachandran. Designing a novel continuous manufacturing plant with superior monitoring and control. European Pharmaceutical Review. 6 Jan 2016.
- Q. Su, Y. Bommireddy, M. Gonzalez, G. V. Reklaitis, Z. K. Nagy. Variation and Risk Analysis in Tablet Press Control for Continuous Manufacturing of Solid Dosage via Direct Compaction. Computer Aided Chemical Engineering. 44:679-684, 2018.
- D. A. Lewis. European Patent Application EP0879140A1. Tablet press monitoring and controlling method and apparatus. Publication date 11/25/1998. https://patents.google.com/patent/EP0879140A1/en (assessed on 06/07/2020)
- D. J. Goodwin, S. van den Ban, M. Denham, I. Barylski. Real time release testing of tablet content and content uniformity. International Journal of Pharmaceutics. 537:183-192, 2018.
- H. Yang, X. Liao, F. Peng, W. Wang, Y. Liu, J. Yan, H. Li. Monitoring of the manufacturing process for ambroxol hydrochloride tablet using NIR-chemometric methods: compression effect on content uniformity model and relevant process parameters testing. Drug Development and Industrial Pharmacy, 41(11):1877-1887,2015.
- V. Pauli, Y. Roggoa, L. Pellegatti, N. Q. N. Trung, F. Elbaz, S. Ensslin, P. Kleinebudde, M. Krumme. Process analytical technology for continuous manufacturing tableting processing: A case study. Journal of Pharmaceutical and Biomedical Analysis. 162:101-111, 2019.
- P. R. Wahl, G. Fruhmann, S. Sacher, G. Straka, S. Sowinski, J. G. Khinast. PAT for tableting: Inline monitoring of API and excipients via NIR spectroscopy. European Journal of Pharmaceutics and Biopharmaceutics. 84:271-278, 2014.
- J. M. Vargas, S. Nielsen, V. Cárdenas, A. Gonzalez, E. Y. Aymat, E. Almodovar, G. Classe, Y. Colón, E. Sanchez, R. J. Romañach. Process analytical technology in continuous manufacturing of a commercial pharmaceutical product. International Journal of Pharmaceutics. 538:167–178, 2018.
- K. Taipale-Kovalainen, A.-P. Karttunen, H. Niinikoski, J. Ketolainen, O. Korhonen. The effects of unintentional and intentional process disturbances on tablet quality during long continuous manufacturing runs. European Journal of Pharmaceutical Sciences. 129:10–20, 2019.
- M. Kuentz. Analytical technologies for real-time drug dissolution and precipitation testing on a small scale. Journal of Pharmacy and Pharmacology. 67:143-159, 2014.
- H. Wu, R.C. Lyon, M.A. Khan, R.J. Voytilla, and J. K. Drennen, III. Integration of Near-Infrared Spectroscopy and Mechanistic Modeling for Predicting Film-Coating and Dissolution of Modified Release Tablets. Industrial & Engineering Chemistry Research. 54(22): 6012–6023, 2015.
- Y. Hattori, M. Sugata, H. Kamata, M. Nagata, T. Nagato, K. Hasegawa, M. Otsuka. Real-time monitoring of the tablet-coating process by near-infrared spectroscopy - Effects of coating polymer concentrations on pharmaceutical properties of tablets. Journal of Drug Delivery Science and Technology. 46:111–121, 2018.
- S. Sacher, P. Wahl, M. Weißensteiner, M. Wolfgang, Y. Pokhilchuk, B. Looser, J. Thies, A. Raffa, J. G. Khinast. Shedding light on coatings: Real-time monitoring of coating quality at industrial scale. International Journal of Pharmaceutics. 566: 57–66, 2019.
- D. Markl & J. A. Zeitler. A Review of Disintegration Mechanisms and Measurement Techniques. Pharmaceutical Research, 34:890–917, 2017.
- R. L. Remmele, Jr., C. Stushnoff, and J.F. Carpenter. Real-time in situ monitoring of lysozyme during lyophilization using infrared spectroscopy: dehydration stress in the presence of sucrose. Pharmaceutical Research, 14(11): 1548-1555, 1997.
- M. Brülls, S. Folestad, A. Sparén & A. Rasmuson. In-Situ Near-Infrared Spectroscopy Monitoring of the Lyophilization Process. Pharmaceutical Research, 20:494–499(2003)
- T.R.M. De Beer, P. Vercruysse, A. Burggraeve, T. Quinten, J. Quyang, X. Zhang, C. Vervaet, J.P. Remon, W.R.G. Baeyens. In-Line and Real-Time Process Monitoring of a Freeze Drying Process Using Raman and NIR Spectroscopy as Complementary Process Analytical Technology (PAT) Tools. Journal of Pharmaceutical Sciences, 98:3430–3446, 2009.
- T. R. M. De Beer, M. Allesø, F. Goethals, A. Coppens, Y. Vander Heyden, H. Lopez De Diego, J. Rantanen, F. Verpoort, C. Vervaet, J. P. Remon, and W. R. G. Baeyens. Implementation of a Process Analytical Technology System in a Freeze-Drying Process Using Raman Spectroscopy for In-Line Process Monitoring. Anal. Chem. 79:7992-8003, 2007.
- D. Fissore, R. Pisano & A. A. Barresi. Process analytical technology for monitoring pharmaceuticals freeze-drying – A comprehensive review. Drying Technology, 36(15):1839-1865, 2018.
- H. Kawasaki, T. Shimanouchi , and Y. Kimura. Recent Development of Optimization of Lyophilization Process. Journal of Chemistry, Volume 2019, Article ID 9502856, 1-14 .
- F. Clementschitsch and K. Bayer. Improvement of bioprocess monitoring: development of novel Concepts. Microbial Cell Factories. 2006; 5: 19
- V. Vojinovic, J.M.S. Cabral, L.P. Fonseca. Real-time bioprocess monitoring. Part I: In situ sensors Sensors and Actuators B 114 (2006) 1083–1091.
- N. R. Abu-Absi, B. M. Kenty, M. E. Cuellar, M. C. Borys, S. Sakhamuri, D. J. Strachan, M. C. Hausladen, Z. J. Li. Real Time Monitoring of Multiple Parameters in Mammalian Cell Culture Bioreactors Using an In-Line Raman Spectroscopy Probe. Biotechnology and Bioengineering, 108(5):1215-1221, 2011.
- Karen A. Esmonde-White, Maryann Cuellar, Carsten Uerpmann, Bruno Lenain, Ian R. Lewis. Raman spectroscopy as a process analytical technology for pharmaceutical manufacturing and bioprocessing. Anal Bioanal Chem (2017) 409:637–649.
- M.-O. Baradez, D. Biziato, E. Hassan, and D. Marshall. Application of Raman Spectroscopy and Univariate Modeling as a Process Analytical Technology for Cell Therapy Bioprocessing. Frontiers in Medicine, 5, Article 47, March 2018.
- K. Buckley and A. G. Ryder. Applications of Raman Spectroscopy in Biopharmaceutical Manufacturing: A Short Review. Applied Spectroscopy 2017, Vol. 71(6) 1085–1116.
- H. Wu, et al., Real Time Monitoring of Bioreactor mAb IgG3 Cell Culture Process Dynamics via Fourier Transform Infrared Spectroscopy: Implications for Enabling Cell Culture Process Analytical Technology. Frontiers of Chemical Science and Engineering. 2015, 9 (3): 386-406.
- S. Goldrick, E. Mercer, G. Montague, D. Lovett, B. Lennox. Control of an Industrial Scale Bioreactor using a PAT Analyser. Proceedings of the 19th World Congress, The International Federation of Automatic Control, Cape Town, South Africa. August 24-29, 2014. Pg. 6222-6227.
- White Paper: Biopharma PAT: Quality Attributes, Critical Process Parameters & Key Performance Indicators at the Bioreactor. May 2018. (accessed on 05/21/2020)
- S. S. Ozturk, J. C. Thrift, J. D. Blackie, D. Naveh. Real-Time Monitoring and Control of Glucose and Lactate Concentrations in a Mammalian Cell Perfusion Reactor. Biotechnology and Bioengineering, 53(4):372-378, 1997.
- C. Cimander, T. Bachinger, C.-F. Mandenius. Integration of distributed multi-analyzer monitoring and control in bioprocessing based on a real-time expert system. Journal of Biotechnology 103:237-248, 2003.
- C. Ündey, S. Ertunc, T. Mistretta, B. Looze. Applied advanced process analytics in biopharmaceutical manufacturing: Challenges and prospects in real-time monitoring and control. Journal of Process Control, 20:1009-1018, 2010.
- N. R Abu-Absi, R. P Martel, A. M Lanza, S. J Clements, M. Borys, & Z. J. Li. Application of spectroscopic methods for monitoring of bioprocesses and the implications for the manufacture of biologics. Pharmaceutical Bioprocessing, 2(3):1-18, 2014.
- C. M.V. Moore. Regulatory Perspective on Real Time Release Testing (RTRT). AAPS Annual Meeting, Washington, DC, 27 Oct 2011.
- S. Chatterjee, 2014. Regulatory Considerations for RTRT (Real Time Release Testing) Based Control Strategy-ONDQA Perspective. AAPS eLearning Webinar.
- M. Jiang, K.A. Severson, J. Christopher Love, H. Madden, P. Swann, L. Zhang, R.D. Braatz. Opportunities and challenges of real-time release testing in biopharmaceutical manufacturing. Biotechnology and Bioengineering. 2017, 114: 2445-2456.
- Juan L. Torres. A Case Study In Real-Time Release Testing. PDA Letter, March 6, 2017.
- L. X. Yu, G. Amidon, M. A. Khan, S. W. Hoag, J. Polli, G. K. Raju, and J. Woodcock. Understanding Pharmaceutical Quality by Design. The AAPS Journal, 16(4):771-783, 2014
- B. N. Singh. Product Development, Manufacturing, and Packaging of Solid Dosage Forms Under QbD and PAT Paradigm: DOE Case Studies for Industrial Applications. AAPS PharmSciTech, 20: 313, 1-49, 2019.
- R. Lakerveld, B. Benyahia, P. L. Heider, H. Zhang, A. Wolfe, C. J. Testa, S. Ogden, D. R. Hersey, S. Mascia, J. M. B. Evans, R. D. Braatz, and P. I. Barton. The Application of an Automated Control Strategy for an Integrated Continuous Pharmaceutical Pilot Plant. Org. Process Res. Dev. 2015, 19, 1088−1100.
- Y. Zhao, J. K. Drennen, S. Mohan, S. Wu, and C. A. Anderson. Feedforward and Feedback Control of a Pharmaceutical Coating Process. AAPS PharmSciTech. (2019) 20: 157.
- R. Singh, F. J. Muzzio, M. Ierapetritou, and R. Ramachandran. A Combined Feed-Forward/Feed-Back Control System for a QbD-Based Continuous Tablet Manufacturing Process. Processes 2015, 3, 339-356.
- N. T. Haas, M. Ierapetritou, R. Singh. Advanced Model Predictive Feedforward/Feedback Control of a Tablet Press. J Pharm Innov (2017) 12:110–123.
- U.K. Singh, B.J.Reizman, S.M.Changl, and J.L.Burt, C. Orella. Reaction Kinetics and Characetrization. Chapter 8. In Chemical Engineering in Pharmaceutical Industry: Active Pharmaceutical Ingredients. 2nd edition. Edited by D.J. Am Ende and M. T. Am Ende. Wiley, 2019, John Wiley & Sons, Inc.
- O. I. Abiodun, A. Jantan, A. B. E. Omolara, K. V. Data, A. M. Umar, O. U. Linus, H. Arshad, A. A. Kazaure, U. Gana, and M. U. Kiru. Comprehensive Review of Artificial Neural Network Applications to Pattern Recognition. IEEE Access, 7: 158820-158846, 2019.
- Srikanth Namuduri et al. Review—Deep Learning Methods for Sensor Based Predictive Maintenance and Future Perspectives for Electrochemical Sensors. 2020 J. Electrochem. Soc. 167(3), 037552.
- M. Z. Alom, T. M. Taha, C. Yakopcic, S. Westberg, P. Sidike, M. S. Nasrin, B. C V. Essen, A. A S. Awwal, and V. K. Asari. The History Began from AlexNet: A Comprehensive Survey on Deep Learning Approaches. https://arxiv.org/ftp/arxiv/papers/1803/1803.01164.pdf. (accessed on 06/10/2020)
- M. I. Razzak, S. Naz and A. Zaib. Deep Learning for Medical Image Processing: Overview, Challenges and Future. https://arxiv.org/ftp/arxiv/papers/1704/1704.06825.pdf. (accessed on 06/10/2020)
- W. Nash, T. Drummond, and N. Birbilis. A review of deep learning in the study of materials degradation. npj Materials Degradation, volume 2, Article number: 37 (2018).
- Y. Bathaee. The Artificial Intelligence Black Box and The Future of Intent and Causation. Harvard Journal of Law & Technology, 31(2):890-938, Spring 2018.
- J. Huang, D. L. Pla. GMP Implementation of Advanced Process Control in Tablet Manufacturing. American Pharmaceutical Review. Tuesday, March 16, 2017.
- J. Markarian. Considering Advanced Process Control for Solid-Dosage Manufacturing. Pharmaceutical Technology. Equipment and Processing Report, 11(3), March 21, 2018.
- S. Romero-Torres, K. Wolfram, J. Armando, S.K. Ahmed, J. Ren, C. Shi, D. Hill, R. Guenard. Biopharmaceutical Process Model Evolution- Enabling Process Knowledge Continuum from an Advanced Process Control Perspective. American Pharmaceutical Review. Tuesday, July 31, 2018.
- P. K. Sarkar. Advanced Process Dynamics and Control. Chapter 13: Control of Unit Operations and Processes. PHI Learning Private Limited. Delhi-110092. 2015. P. 641-702.
- A. Gupta, A. Giridhar, V. Venkatasubramanian, and G. V. Reklaitis. Intelligent Alarm Management Applied to Continuous Pharmaceutical Tablet Manufacturing: An Integrated Approach. Industrial & Engineering Chemistry Research. 2013, 52, 12357-12368.
- D.C. Montgomery. Introduction to Statistical Quality Control, 8th Edition. Wiley & Sons, Inc, 2020.
- K. D. Seibert, S. Sethuraman, J. D. Mitchell, K. L. Griffiths, B. McGarvey. The Use of Routine Process Capability for the Determination of Process Parameter Criticality in Small-molecule API Synthesis. J Pharm Innov (2008) 3:105–112.
- L. Xie, H. Wu, M. Shen, L. Augsburger, R.C. Lyon, M.A. Khan, A.S. Hussain, and S.W. Hoag. Quality-by-Design (QbD): Effects of Testing Parameters and Formulation Variables on the Segregation Tendency of Pharmaceutical Powder Measured by the ASTM D 6940-04 Segregation Test. Journal of Pharmaceutical Sciences 97(10):4485-97, 2008
- R. A. Lionberger, S. L. Lee, L. Lee, A. Raw, and L. X. Yu. Quality by Design: Concepts for ANDAs. The AAPS Journal, 10(2):268-276, June 2008.
- M. George, I. Ghosh. Identifying the correlation between drug/stabilizer properties and critical quality attributes (CQAs) of nanosuspension formulation prepared by wet media milling technology. European Journal of Pharmaceutical Sciences 48: 142-152, 2013.
- N. Alt, T.Y. Zhang, P. Motchnik, R. Taticcek, V. Quarmby, T. Schlothauer, H. Beck, T. Emrich, R.J. Harris. Determination of critical quality attributes for monoclonal antibodies using quality by design principles. Biologicals 44:291-305, 2016.
- P. Hamilton, M. J. Sanganee, J. P. Graham, T. Hartwig, A. Ironmonger, C. Priestley, L. A. Senior, D. R. Thompson, and M. R. Webb. Using PAT to Understand, Control, and Rapidly Scale Up the Production of a Hydrogenation Reaction and Isolation of Pharmaceutical Intermediate. Org. Process Res. Dev. 2015, 19, 236−243.
- Sartorius. Reducing upstream processing scale-up risks using QbD-enabling platforms and PAT tools. Manufacturing Chemist. Equipment. 8-Jan-2017 (https://www.manufacturingchemist.com/news/article_page/Reducing_upstream_processing_scaleup_risks_using_QbD-enabling_platforms_and_PAT_tools/124240) (accessed on 06/12/2020)
- H. Wu, K. Sowrirajan, M. Jaiqirdar. Applying Dynamic Similarity Principles to a Narrow Therapeutic Index Drug Powder Blending Process Scale-up: Laboratory Case Studies and Relevant Regulatory Experience. 2018 AIChE Annual Meeting, Pittsburgh, PA. Oct 29-Nov 2, 2018.
- G. A. Van Buskirk, et al., Best Practices for the Development, Scale-up, and Post-approval Change Control of IR and MR Dosage Forms in the Current Quality-by-Design Paradigm. AAPS PharmSciTech, 15(3):665-693, June 2014.
- O. F. Valilai, M. Houshmand. A collaborative and integrated platform to support distributed manufacturing system using a service-oriented approach based on cloud computing paradigm. Robotics and Computer-Integrated Manufacturing. 29 (2012):119-127.
- C. Hays. Computer Integrated Manufacturing System-Advantages of connected factory floor assets. Purdue WHIN Workshop Series for Digital Tools for Manufacturing, Nov 20, 2019.
- Alexey Dudoladov. Chapter 10, Manufacturing Engineering. Exploring Engineering, Third Edition. 2013.
- J. Odum. Design drivers for flexible pharma manufacturing assets. Part I of the series: The future of flexible manufacturing. TechTalk powered by nne. Flexible Manufacturing Systems. (accessed on 06/14/2020)
- E. S. Langer, R. A. Rader. Biopharmaceutical Manufacturing is Shifting to Single-Use Systems. Are the Dinosaurs, the Large Stainless Steel Facilities, Becoming Extinct? American Pharmaceutical Review, October 23, 2018.
- R. F. Meyer, Y. Kapoor. The Case for Flexibility in Pharmaceutical Manufacturing. American Pharmaceutical Review. October 4, 2019.
- Bedir Tekinerdogan, Alp Oral. Chapter 8 - Performance Isolation in Cloud-Based Big Data Architectures. in Software Architecture for Big Data and the Cloud. Pages 127-145, 2017.
- H. Zou and T. Hastie. Regularization and Variable Selection via the Elastic Net. Journal of the Royal Statistical Society: Series B (Statistical Methodology). 67, Part 2, pp. 301-320, 2005.
- S. Chakrabortya, M. Ghoshb, B. K. Mallick. Bayesian nonlinear regression for large p small n problems. Journal of Multivariate Analysis, 108: 28-40, 2012.
- R. Tibshirani. Regression shrinkage and selection via the lasso. Journal of the Royal Statistical Society: Series B (Statistical Methodology). 58(1): 267–288, 1996.
- P. Pawar, S. Talwar, D. Reddy, C. Bandi, H. Wu*, K. Sowrirajan, R. Friedman, G. Drazer, J. K. Drennen, III, F. J. Muzzio. A “Large-N” Content Uniformity Process Analytical Technology (PAT) Method of Phenytoin Sodium Tablets. Journal of Pharmaceutical Sciences. 108(1):494-505, 2019.
- V. Steinwandter, D. Borchert, and C. Herwig. Data science tools and applications on the way to Pharma 4.0. Drug Discovery Today, 24(9):1795-1805, 2019
- M. Mc Namara, M. Gadsby. Integrating the OSIsoft PI System with synTQ for High Performance, Low Maintenance PAT Method Integration. OSIsoft EMEA User Conference 2017, London. (accessed on 05/06/2020)
- M. Morrow and B. Zhang. It’s the Data Stupid: How PI AF Analytics Rescued Our Realtime Multivariate Process Monitoring, OSIsoft PI World Conference, San Francisco, 2019. (accessed on 05/06/2020)
- D. Markl et al., Supervisory Control System for Monitoring a Pharmaceutical Hot Melt Extrusion Process. AAPS PharmSciTech, Vol 14, No.3, Sept 2013, p.1034-1044.
- FDA Guidance for Industry. Q7 Good Manufacturing Practice Guidance for Active Pharmaceutical Ingredients. September 2016. (assessed on 05/21/2020)
- FDA Guidance for Industry (Draft): Development and Submission of Near Infrared Analytical Procedures. March 2015. (accessed on 05/26/2020)
- ASTM E55.01 on Process Understanding and PAT System Management, Implementation and Practice. (assessed on 06/15/2020)
- ASTM E 55. Revision of E2891 - 13 Standard Guide for Multivariate Data Analysis in Pharmaceutical Development and Manufacturing Applications. (accessed on 05/26/2020)
Author Biography
Dr. Huiquan Wu received his Ph.D. in Chemical Engineering with a minor in Materials Science and Engineering from Iowa State University in 2001. Dr. Wu worked as a senior process development engineer at LSI Logic and ACM Research from March 2001 to August 2002. Since joining FDA in August 2002, Dr. Wu has been worked as a visiting associate (August 2002-March 2005), staff fellow (March 2005-December 2011), and research chemical engineer (January 2012-present) at various quality areas within the Center for Drug Evaluation and Research (CDER), Food and Drug Administration (FDA), including Office of Pharmaceutical Sciences (OPS) PAT Policy and Research Development Team (August 2002-March 2005), Office of Testing and Research (OTR) Division of Product Quality Research (DPQR) (March 2005-Juanary 2015), Office of Process and Facilities (OPF) Division of Process Assessment 1(DPA 1) (January 2015-October 2019), and then Office of Pharmaceutical Manufacturing Assessment (OPMA) Division of Pharmaceutical Manufacturing I (DPM I) (October 2019-present). Since joining OPF/ OPMA in January 2015, Dr. Wu has been conducted primary regulatory and scientific assessment of NDAs/ANDAs/INDs/NDA Supplements/ANDA Supplements. (NDA: New Drug Application; ANDA: Abbreviated New Drug Application; IND: Investigational New Drug Application).