Philippe Moingeon, PhD, MBA Head of Immunoinflammation Research and Development, Servier, Cedex, France Académie nationale de Pharmacie, Paris, France
Abstract
Artificial Intelligence (AI) is a convergence of technologies which recapitulates four dimensions of human intelligence, i.e., sensing, thinking, acting and learning. AI is being applied to revolutionize drug discovery and development by making this costly and complex process ultimately cheaper and more effective. It works with Machine Learning (ML) to capture the value of massive and multimodal data to build up predictive models supporting decision-making along drug development. AI is being used to model disease heterogeneity, identify therapeutic targets, design and optimize drug-candidates and predict in silico their clinical efficacy. As such, it will significantly shorten the discovery phase and reduce failure rates during drug development by strengthening the rationale behind the selection of target and drug candidates. AI brings an unprecedented level of knowledge to both the patient specificities and the attributes of drug candidates, thereby paving the ground for Computational Precision Medicine. The latter enables drug developers precisely tailor therapies to the characteristics of individual patients in terms of their physiology, the pathophysiology of their disease and their susceptibilities to genetic and environmental risks.
Keywords
Artificial intelligence, drug development, precision medicine.
Why is AI Raising Tremendous Interest in Pharma and Biotech Industries?
Drug development requires as of today on average 12 years of development and a $2.6B investment for each individual drug eventually made available to the patient.1 The complexity of new drug development has been in part due to growing regulatory requirements in the last decade to demonstrate the efficacy and safety of therapeutic molecules. It is also associated with the need to prove a superior efficacy to existing drugs, a concern termed the “Better than the Beatles problem”. More recently, the importance of taking better-educated decisions, for example when selecting the therapeutic target or the drug candidate has also been emphasized.2 For those reasons combined the return on investment of each dollar spent in R and D in support of drug development has been continuously declining over the years (Figure 1A). A very high attrition rate is observed in the course of drug evaluation, with an estimated 6.2% of drugs selected in the discovery phase eventually reaching patients.3 Whereas this attrition is observed all along the development of a new drug, the failure rate is most particularly high at the end of Phase 2 studies, i.e. when significant costs have already been incurred (Figure 1B).
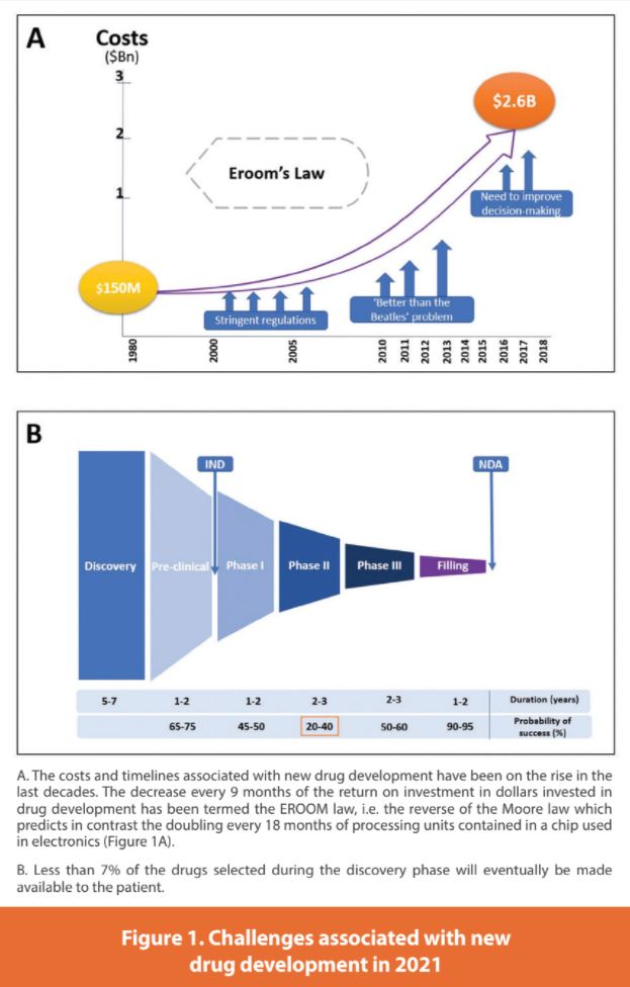
In this context, the availability of AI-based predictive modeling provides the opportunity to both speed up and increase the success rate of new drug development.4-6 AI aims to develop intelligent machines inspired by primate brain physiology, by using a convergence of technologies related to sensing, thinking, acting and learning (Table 1).7,8 In light of recent advances in machine learning, AI can dramatically outperform conventional bioinformatics by integrating massive amounts of multi-modal data, both structured and unstructured in order to create probabilistic and dynamic models of a problem (Table 1).6,8 Such models can then help humans to make appropriate decisions and predict the future.
As it applies to drug design and development, AI can be used to integrate specific sets of data and create predictive models in order to inform multiple important decisions. The latter include the choice of a relevant therapeutic target and of the right drug candidate, the selection of proper dosing and administration regimens, as well as the inclusion of patients with specific requisites to document drug efficacy in clinical studies.4-6
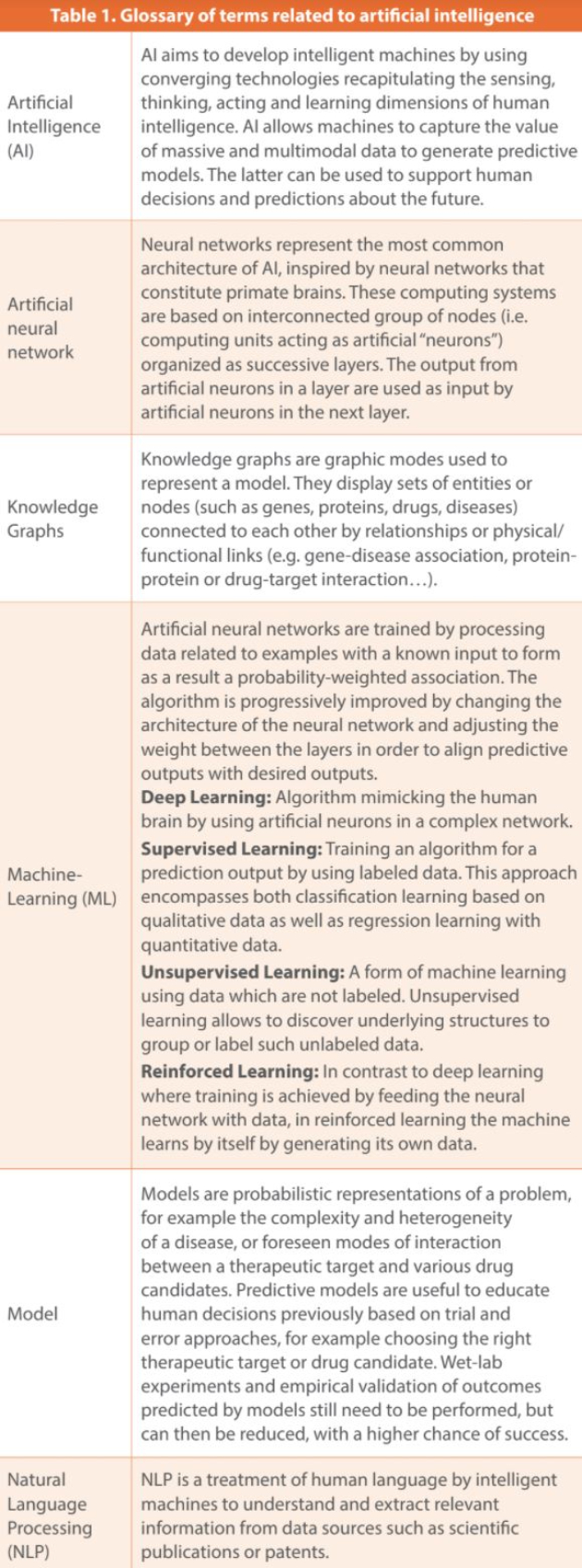
Applications of AI to Drug Discovery and Development
Schematically, four categories of applications of AI to drug discovery and development can be considered, including (i) the production of disease models based on molecular profiling data from patients, (ii) the identification of candidate therapeutic targets within main dysregulated molecular pathways, (iii) the selection and optimization of drug candidates interacting with such targets, and (iv) the in silico evaluation of clinical efficacy (Figure 2).
Disease models can be generated following extensive molecular profiling of large cohorts of patients. The latter is commonly achieved by applying multi-omics technologies (i.e., genomics, epigenetics, transcriptomics, proteomics, metabolomics, deep immunophenotyping...) to the characterization of blood and/or tissue samples. Patients can then be stratified as homogeneous sub-groups by integrating these comprehensive biological data together with detailed clinical information regarding the progression and severity of their disease or their response to treatments.9-11 To further reflect disease heterogeneity, gene enrichment analyses are conducted within each patient subgroup to identify molecular pathways either up-or down-regulated when compared with healthy controls. This results in a classification of patients in sub-groups associated with distinct endotypes, i.e. underlying pathophysiological mechanisms.6,10 As an advantage over previously used clinical phenotypes, such a stratification in endotypes better supports a precision medicine approach aiming to treat well-defined subgroups of patients with therapies tailored to their precise biological and clinical specificities.
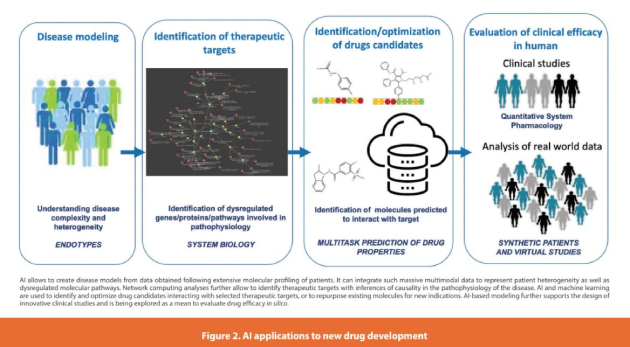
Specific network computational analyses are conducted to represent the disease in the blood and/or target organs as a biological system assembling dysregulated genes or proteins. Key disease-associated genes including master regulators can then be identified, which are predicted to be involved in the causality of the disease.12 The latter obviously can be considered as valid candidate therapeutic targets. To further prioritize those targets for further investigation, additional characteristics beyond biological relevance to the disease are documented, including druggability, potential safety issues when interfering with this target, or innovativeness documented by Natural Language Processing (NLP) mining of patent libraries. Noteworthy, recent advances in 3D-structure modeling, most particularly through the prediction of protein structures from primary amino-acid sequences by DeepMind’s AlphaFold algorithm now provide highly relevant information to select drug candidates foreseen to interact with those targets.13
Beyond Quantitative Structure-Activity Relationships (QSAR) analyses performed by computational chemistry, machine learning is commonly applied to train artificial neural networks on ligand-based virtual screens to identify and optimize drugs interacting with candidate therapeutic targets.14-16 In addition to extending the explored chemical space to millions of molecules, in silico methods further allow multi-task parallel prediction of properties associated with selected drug candidates. The latter encompass not only their pharmacological activity but also their ADMET (absorption, distribution, metabolism, excretion and toxicity) properties.16,17 Deep learning approaches and the use of Generative Adversarial Networks are also implemented to perform de novo drug design and optimization, or even conduct retrosynthesis analyses to document the chemical synthesis of those innovative molecules. Network-based proximity analyses to predict drug-target interactions are also used to repurpose existing drugs into new indications.15
The application of AI in support of clinical studies has recently generated considerable interest. AI can facilitate patient selection in the trials by integrating biological, medical imaging and clinical data documenting the patient’s specificities. Automated mining with NLP of Real-World Evidence data or health records can further be used to assess patient eligibility in clinical studies, when they fulfill the inclusion criteria. AI is used during trial monitoring to capture, share and analyze remotely patient-reported measurements and outcomes generated by wearable sensors or devices. In a different application particularly useful to evaluate drug candidates in life-threatening or rare disease indications, it is also applied to design virtual placebo groups comprising “synthetic” patients.18,19 The latter are real patients who fulfill the inclusion/exclusion criteria but do not participate in the trial, and whose evolution under standard of care is modeled from real-world evidence data. Another exciting frontier will consist of predicting the efficacy of an investigational drug from virtual clinical studies. To this aim, Quantitative System Pharmacology (QSP) models of a disease are currently built up from data related to biological processes occurring in the blood or in tissues in association with clinical symptoms.20 The interactions between components of this biological system as well as the main characteristics of the drug candidate (e.g. affinity for the target, pharmacokinetics and biodistribution) are then computed as mathematical differential equations to predict in silico how this drug could alleviate symptoms. QSP models can also evaluate different dosing and administration regimens, select clinical endpoints to use in confirmatory real-world trials and identify biomarkers to monitor patients. In addition, AI-generated models of diseases in the form of interactomes of dysregulated genes or proteins with inferences of causality in the pathophysiology are currently being explored to test the impact of in silico perturbation of candidate therapeutic targets on the disease system.
Challenges and Points to Consider
When applying AI to drug design and development, accessing data with proper quality is paramount. Data management is thus critical for any organization in terms of curation, governance and respect of regulations.6,21 FAIR guiding principles have been established to promote the findability, accessibility, interoperability, reusability and exchange of data. The US Health Information Technology for Economic and Clinical Act and the European General Data Protection Regulation(GDPR) further impose that specific data governance practices are implemented with regard to the use, storage and sharing of personal health data, considered as highly sensitive and confidential.6
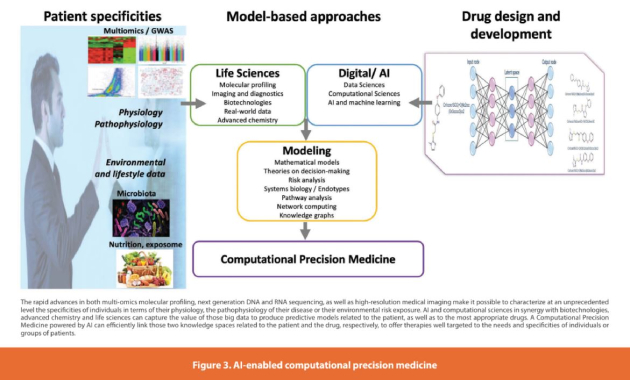
In order for the health industry to fully capitalize on the power of AI, it is also important to ensure that proof of evidence originating from computer models are accepted by regulatory authorities such as the FDA or EMA. Besides a necessary dialog with regulators when implementing those technologies, inferences made from AI-powered models need obviously to be validated by empirical data generated in the laboratory or during clinical studies. Candidate therapeutic targets may for example be confirmed by CRISPR-Cas9 gene deletion or siRNA silencing experiments. Predicted drug candidates will still need to be synthesized and tested in human cellular assays or animal models. Similarly, some real-world confirmatory clinical studies will have to be conducted to confirm drug efficacy. Nevertheless, those required real-world studies will be fewer, simpler and better designed with the help of AI.
The growing interface between human and intelligent machines will represent in the years to come a major challenge. Specifically, up to 90% of health-related jobs are anticipated to necessitate solid digital skills by 2040.22 Whereas many health care professionals (HCPs) are worried about being replaced in their jobs by intelligent machines, AI should positively complement and enhance the broad range of human expertise required for drug design and development. Human judgement will obviously remain critical to assess and validate computational outcomes, but also to foresee potential ethical implications when implementing AI. In this regard, the ultimate responsibility of diagnostic or therapeutic decisions informed by algorithms will still lie for decades with HCPs. Thus, training and education will be needed to facilitate the acculturation to AI and digital technologies of professionals across academia and the health industry. Establishing a true convergence of human and artificial intelligences will likely represent a key success factor in the implementation of AI to transform ways of working.23
The Promise of a Computational Precision Medicine
The health industry is currently integrating AI in support of drug development at a fast pace. Without any doubt, AI will make the process of drug design and development faster and much more efficient. As a result, both cost and timelines associated with drug discovery will be substantially reduced. Recently, three precision-engineered small molecule drugs have been generated by AI in the fields of immuno-oncology and neurological diseases by Exscientia in collaboration with Sumitomo Dainippon. Some of these new drugs have now entered Phase I clinical evaluation after only 12 months of research, to be compared to the five to seven years of discovery usually needed to bring a new drug candidate into clinical testing. A new broad-spectrum antibiotic named Halicin has been identified following AI-mining of existing molecules, with many other examples of drugs proposed by network computing for repurposing to treat cancers, neurological diseases or COVID-19.24 Beyond synthetic chemical molecules, machine learning is further being applied to train neural networks to design and select biologicals with predicted pharmacological properties, including monoclonal antibodies, recombinant proteins and peptides. Model-based approaches powered by AI will also significantly increase the probability of success during late development in light of a much stronger rationale for selecting the therapeutic target and drug candidate prior to entering clinical development. Virtual clinical studies should also in the mid-term facilitate the evaluation of drug efficacy, thus significantly decreasing the costs and duration of clinical development.
As a result, we can predict in the forthcoming five to ten years a significant increase in numbers of innovative drugs made available to the patients. This trend will be paralleled by an unprecedented knowledge of patients, driven by AI-based upon massive biological, clinical, but also environmental data documenting the specificities of their physiology, disease, and lifestyle. Consequently, AI will foster Computational Precision Medicine through the continuous update of multiple predictive models encompassing the patient, the disease and the drug candidate (Figure 3). As such, it will allow across all disease areas to propose rationally designed treatments and combination therapies targeted to the specificities of individual patients or well-defined homogeneous subgroups. This computational precision medicine will further predict individual responses to therapy. It will also engage patients and healthy individuals to manage their health on the basis of their predicted exposure to genetic and environmental risks, thus promoting health and well-being measures to ensure disease prevention, early detection and treatment.
References
- DiMasi JA, Grabowski HG, Hansen RW. Innovation in the pharmaceutical industry: New estimates of R&D costs. J Health Econ. 2016;47:20-33. doi:10.1016/j.jhealeco.2016.01.012
- Ringel MS, Scannell JW, Baedeker M, Schulze U. Breaking Eroom’s Law. Nature Reviews Drug Discovery. 2020;19(12):833-834. doi:10.1038/d41573-020-00059-3
- Waring MJ, Arrowsmith J, Leach AR, et al. An analysis of the attrition of drug candidates from four major pharmaceutical companies. Nat Rev Drug Discov. 2015;14(7):475-486. doi:10.1038/nrd4609
- Vamathevan J, Clark D, Czodrowski P, et al. Applications of machine learning in drug discovery and development. Nat Rev Drug Discov. 2019;18(6):463-477. doi:10.1038/ s41573-019-0024-5
- Paul D, Sanap G, Shenoy S, Kalyane D, Kalia K, Tekade RK. Artificial intelligence in drug discovery and development. Drug Discov Today. 2021;26(1):80-93. doi:10.1016/j. drudis.2020.10.010
- Moingeon P. Artificial intelligence and the future of medicine: a multidimensional analysis. Life Res. 2021; 4 (1): 3-10. doi:10.12032/ Life2021-0103-301
- Ullman S. Using neuroscience to develop artificial intelligence. Science. 2019;363(6428):692-693. doi:10.1126/science.aau6595
- LeCun Y, Bengio Y, Hinton G. Deep learning. Nature. 2015;521(7553):436-444. doi:10.1038/ nature14539
- García Del Valle EP, Lagunes García G, Prieto Santamaría L, Zanin M, Menasalvas Ruiz E, Rodríguez-González A. Disease networks and their contribution to disease understanding: A review of their evolution, techniques and data sources. J Biomed Inform. 2019;94:103206. doi:10.1016/j.jbi.2019.103206
- Hasin Y, Seldin M, Lusis A. Multi-omics approaches to disease. Genome Biology. 2017;18(1):83. doi:10.1186/s13059-017-1215-1
- Tini G, Marchetti L, Priami C, Scott-Boyer M-P. Multi-omics integration-a comparison of unsupervised clustering methodologies. Brief Bioinform. 2019;20(4):1269-1279. doi:10.1093/bib/bbx167
- Barabási A-L, Gulbahce N, Loscalzo J. Network medicine: a network-based approach to human disease. Nat Rev Genet. 2011;12(1):56-68. doi:10.1038/nrg2918
- Senior AW, Evans R, Jumper J, et al. Improved protein structure prediction using potentials from deep learning. Nature. 2020;577(7792):706-710. doi:10.1038/s41586-019-1923-7
- Guney E, Menche J, Vidal M, Barábasi A-L. Network-based in silico drug efficacy screening. Nat Commun. 2016;7(1):10331. doi:10.1038/ncomms10331
- Cheng F, Desai RJ, Handy DE, et al. Network-based approach to prediction and population- based validation of in silico drug repurposing. Nat Commun. 2018;9(1):2691. doi:10.1038/ s41467-018-05116-5
- Rifaioglu AS, Atas H, Martin MJ, Cetin-Atalay R, Atalay V, Doğan T. Recent applications of deep learning and machine intelligence on in silico drug discovery: methods, tools and databases. Brief Bioinform. 2019;20(5):1878-1912. doi:10.1093/bib/bby061
- Wieder O, Kohlbacher S, Kuenemann M, et al. A compact review of molecular property prediction with graph neural networks. Drug Discovery Today: Technologies. December 2020. doi:10.1016/j.ddtec.2020.11.009
- Shah P, Kendall F, Khozin S, et al. Artificial intelligence and machine learning in clinical development: a translational perspective. npj Digit Med. 2019;2(1):1-5. doi:10.1038/ s41746-019-0148-3
- Tucker A, Wang Z, Rotalinti Y, Myles P. Generating high-fidelity synthetic patient data for assessing machine learning healthcare software. npj Digit Med. 2020;3(1):1-13. doi:10.1038/s41746-020-00353-9
- Friedrich CM. A model qualification method for mechanistic physiological QSP models to support model-informed drug development. CPT Pharmacometrics Syst Pharmacol. 2016;5(2):43-53. doi:10.1002/psp4.12056
- Eberendu A. Unstructured Data: an overview of the data of Big Data. International Journal of Computer Trends and Technology. 2016;38:46-50. doi:10.14445/22312803/IJCTT- V38P109
- The Topol Review — NHS Health Education England. The Topol Review — NHS Health Education England. https://topol.hee.nhs.uk/. Accessed August 30, 2021.
- Topol EJ. High-performance medicine: the convergence of human and artificial intelligence. Nat Med. 2019;25(1):44-56. doi:10.1038/s41591-018-0300-7 24. Stokes JM, Yang K, Swanson K, et al. A Deep Learning Approach to Antibiotic Discovery. Cell. 2020;180(4):688-702.e13. doi:10.1016/j.cell.2020.01.021
Author Biography
Philippe Moingeon received his PhD in Immunology from Paris XI University and an MBA from Open University Business School (UK). As a former Assistant Professor at Harvard Medical School (Boston, USA), he specialized in the development of biologicals and immunotherapies, holding several leadership positions in the vaccine and pharmaceutical industries.
His research interests encompass artificial intelligence applications to drug development, medicine of the future and technological evolution of mankind.
Subscribe to our e-Newsletters
Stay up to date with the latest news, articles, and events. Plus, get special offers
from American Pharmaceutical Review – all delivered right to your inbox! Sign up now!