Lipinski’s seminal paper on experimental and computational (in silico) methods to estimate the solubility and permeability of drug candidates was published just over 15-years ago [1]. The iconic ‘Rule of 5’ predicted that absorption was adversely impacted when the calculated LogP (cLogP) was greater than 5, when molecular weight (MW) was greater than 500, when there are greater than 5 H-bond donors or greater than 10 H-bond acceptors. The related concept of ‘drug-likeness’ importantly focuses on both potency and physicochemical attributes, using tools such as lipophilic efficiency [2] or ligand efficiency [3].
Drug-likeness and related concepts have been widely-used across the pharmaceutical industry to attempt to reduce the very high attrition rates currently seen with unprecedented pharmacological targets. Unfortunately, both combinatorial chemistry and high throughput screening (HTS) tends to favor leads with higher MW, higher cLogP and lowered solubility [1].
Therefore, successful drug discovery strategies appear to be a balance between trying to optimize both ‘hydrophobicity-driven potency and hydrophilicity-driven biopharmaceutics properties’ [4, 5]. Consequently, an over-reliance on optimizing potency to the detriment of physicochemical properties will yield sub-optimal ADMET (absorption, distribution, metabolism, excretion and toxicity) properties and reduce the likelihood of clinical success [6]. However, the lack of optimal physicochemical attributes can often be offset using sophisticated formulation strategies [7, 8], and deficiencies in these properties can often be rate limiting to the progression of drug candidates. Hence, computational methods that can qualitatively predict physicochemical properties, e.g. solubility, before a compound is synthesized based on molecular structure, are an essential requirement of drug discovery.
In this article, the authors will provide an overview of approaches to predict and measure solubility in the pharmaceutical R&D environment with the goal of providing relevant information at the appropriate stage of the process.
Relationships Between Solubility and Physicochemical Properties
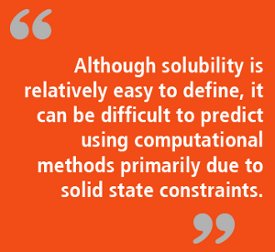
There are significant numbers of computational methods reported within the literature for predicting solubility from underlying molecular properties. These include electronic and topological evaluations, hydrophilicity/hydrophobicity assessments, molecular surface area calculations, etc [9]. In addition, other researchers have attempted to predict solubility using fragment-based models [10], with the three most favored approaches being computational models utilizing molecular topology [11], group contribution approaches [12] and E-state indices [13]. However, these computational methods need to be able to cope with significant numbers of compounds and filter-out ‘non drug-like’ compounds. The methods must help to focus chemistry initiatives on programs with improved physicochemical attributes, thereby enhancing productivity. Importantly, it should be clearly understood that early discovery methodologies provide qualitative and not quantitative outcomes [14].
The challenges inherent in solubility prediction were graphically highlighted by the recent Solubility Challenge. An academic research group [15] measured the equilibrium solubilities of 100 drug-like molecules at fixed temperature and ionic strength. Using this training dataset, they requested other research groups to predict, using their own preferred computational approach(es), the intrinsic solubilities of a further 32 drug-like molecules. The training set was selected to represent a wide range of chemical space with MW ranging from 115 (proline) to 645 (amiodarone), that had pKas between 1 and 12. The intrinsic solubilities spanned over 7-orders of magnitude from the poorly soluble (amiodarone) to the highly soluble (acetaminophen), with a relatively even spread of intermediate values.
The authors received 100+ entries to the Solubility Challenge [16]. Participants used the entire spectrum of available computational tools and this challenge therefore provided a holistic overview of our ability to predict aqueous solubility. The authors could not recommend the best approach(es), rather a number of methodologies that were equally successful at predicting aqueous solubility were identified. Some participants were surprised that the simple models were superior to the more complex methodologies [17]. Faller and Ertl [10] went further claiming that the advantages of the complex models where debatable when compared with the simple cLogP correlations [18]. Hewitt et al. [17] indicated that data quality was pivotal to successful predictivity and even with the ‘high quality’ dataset provided in the ‘Challenge’, questions were still raised about data quality, and it is critical to understand the ‘applicability domain‘ (i.e. the chemical space where the model works best). Understandably, predictions made outside of this domain will be less reliable. Despite the impressive size of some paticipants’ training sets, (46,000 compounds of known solubility), their methods still performed poorly for both soluble and insoluble compounds [19]. Interestingly, Kramer et al. [20] showed improved solubility predictions with their metaClassifier approach, despite the fact that their training set was based on kinetic rather than equilibrium solubilities. They showed a high prediction accuracy for solubility (77.8%), but typically showing a high bias, possibly because their training set used small levels of DMSO as a co-solvent and were measured at pH 7.4. However, their model correctly predicted only one-third of the insoluble compounds in the dataset. Finally, the accuracy of the in-silico model needs to be greater than that of the experimental determinations [10].
The biggest obstacle to accurate solubility prediction is still the unpredictable nature of the solid-state (polymorphs, solvates, salts, hydrates, co-crystals, amorphous, etc.) [21], and how to effectively model enthalpy and entropy of the system, i.e. moving from an ordered, structured low entropy solid state to a disordered, unstructured high entropy solution state. So far polymorphs still cannot be reliably predicted [22] and accordingly their effect on solubility cannot be predicted by in-silico tools.
Yalkowski et al. [23] derived the General Solubility Equation (GSE), to try and better model solubility:
LogS = -LogP -0.01 * (MPt – 25) + 0.5 (Eq. 1)
where S is the intrinsic solubility, P is the octanol/water partition coefficient and MPt is the melting point
However, the MPt term is only partially successful in addressing solid state considerations and the impact on aqueous solubility. It is also evident from the GSE that logP is the major variable in the equation 1 [24]. Indeed, medicinal chemists can more easily influence logP than MPt (more difficult to predict or control and as a consequence MPt is not typically measured) and therefore optimizing logP tends to be the main focus in some discovery organizations. Typical marketed drugs have cLogPs of 2.5 and it is probably not a coincidence that this also corresponds to the upper limit of good solubility as predicted by the GSE [25]. Unfortunately, poor aqueous solubility is therefore the logical outcome of introducing overly hydrophobic character into potential drug candidates.
The GSE constraint of clogP of > 2.5 is probably the worst case scenario as it does not reflect the positive impact that ionization can have in improving aqueous solubility, therefore replacing LogP with LogDpH 7.4, produces a more predictive GSE:
LogSpH 7.4 = -LogDpH 7.4 -0.01 * (MPt – 25) + 0.5 (Eq. 2)
Hill and Young [24] evaluated a large dataset (ca. 20,000 compounds), where measured LogDpH 7.4, together with calculated values for hydrophobicity (cLogP and clogD7.4), accurate kinetic solubility measurements (at pH 7.4), MW and the number of aromatic rings were all available. The authors showed marked differences between measured and calculated hydrophobicity with compounds of decreasing solubility. Poorly soluble compounds (< 30 μM) show particularly bad correlations (R2 = 0.11), this improves slightly (R2 = 0.32) as solubility increases (30- 200 μM), with the ‘best’ correlation occurring with good solubility compounds (>200 μM) compounds (R2 = 0.462). Interestingly, these data support the contention that calculated LogD7.4 (or cLogP) might be a better predictor of hydrophobicity rather than using the measured value [24].
Recently, the negative impacts of aromaticity on solubility have been reported. These include the number of aromatic rings [26, 27], the aromatic portion (AP) [9] and the percentage of sp3 hybridized atoms [28] within the target molecule. Molecules with low lipophilicity are more likely to display poor solubility arising from solid state considerations, i.e. ‘brick dust molecules’; whereas highly lipophilic compounds are solubility limited due to inadequate solvation, i.e. ‘grease ball molecules’ [29]. Various scenarios were modeled and showed that for compounds with a MPt of > 250°C and cLogP of > 2, the GSE demonstrates that solidstate considerations will predominate (over 50%); whereas, when the cLogP is increased beyond 6, that solid-state considerations drop markedly (about 25%). Thus, planar, flat and rigid molecules with ring systems have a high likelihood (86%) of demonstrating low aqueous solubility [29]. How molecular planarity reduces solubility and how solubility can be increased by disrupting planarity has been evaluated by Ishikawa [30]. This can be explained by the increased lattice energy (and MPt) owing to enhanced π- π stacking of the planar aromatic systems. Hill and Young [24] also demonstrate enhanced correlations between the number of aromatic ring systems and cLogDpH 7.4 (as opposed to LogP) and ultimately solubility. They proposed a solubility forecast index (SFI):
SFI = cLogDpH 7.4 + number of aromatic rings (Eq. 3)
Where SFI < 5, then there is typically good aqueous solubility and they contended that each aromatic ring system is equivalent to an extra log unit of cLogDpH 7.4. The average number of aromatic ring systems in marketed oral products is 1.6 [23] and thus the average SFI would be 2.4.
Approaches to Measuring Solubility during Different Phases of Research and Development
After the compound has been synthesized and is physically available, solubility will be measured for the first time. Concepts and workflows to obtain measured solubility at this stage vary from organization to organization as in comparison to in-silico assessment: the number of compounds for which solubility is assessed largely determines the amount of work. Solubility could be measured for every compound which is freshly obtained or might be measured upon request. However, at this stage it is clear that solubility has to be measured for a very large number of compounds and therefore very efficient procedures have to be in place. There are two main purposes of measuring solubility at this stage [31]:
- First, solubility provides a means to answer the fundamental question: is the compound dissolved in the assay medium or has it precipitated out if results from other assays are problematic and need to be questioned? This query becomes relevant for many types of assays, e.g. biochemical and cellular assays that demonstrate activity of the compound. The same question also applies to assays that support safety testing which have been shifted more and more towards earlier stages of research during recent years. In this case, low solubility of a compound might yield false negatives for the respective assay and consequently hide safety related risks of a compound or a whole series or scaffold(s).
- Secondly, even at this early stage solubility will be used as one of many parameters relevant for compound optimization. The final goal should be envisaged to deliver compounds with sufficient solubility to realize high bioavailability and to simplify formulation development and clinical progression.
From a technical standpoint, realizing the required throughput to fulfill both objectives requires a high degree of automation. The key to this – as for many other assay formats – is provided by using pre-dissolved compounds. Typically, 10 mmol solutions in DMSO are utilized. This avoids handling of the solid material which might not always be crystalline but could be oily, sticky or highly electrostatic. Instead of weighing the compounds, compound handling can be carried out by simple volumetric dispensing, i.e. pipetting steps. Accordingly, it becomes feasible to implement solubility determinations on robotic systems which carry out manipulation such as volumetric dispensing, compound precipitation and solidliquid phase separation by filtration or centrifugation. Typically, these liquid handling systems which carry out the volumetric dispensing and related activities can be combined with highly sensitive analytical systems, e.g. HPLC or UPLC utilizing generic methodologies and can be applied to automated solubility assessments with throughputs of 10-100 of compounds per day [32-36].
However, one has to bear in mind that this type of kinetic solubility does not answer the question, “to what extent does my compound dissolve?”, but instead provides the answer to the related question, “to what extent does my compound precipitate?”. As most drugs are intended for oral administration, the first question is more relevant during later research phases. The key differentiating point between kinetic solubility – which is obtained using the pre-dissolved compound – and thermodynamic solubility – which is obtained using the solid compound – is given by the fact the kinetic solubility will refer in many cases to the amorphous phase. In a kinetic solubility assay, the compound will have only very limited time to precipitate out and accordingly will be mainly amorphous in nature. As a consequence, solubility will be significantly enhanced compared to thermodynamic solubility (which typically utilizes the crystalline phase) [37].
The main – and in many cases only – difference between kinetic and thermodynamic solubility assays is the usage of solid compound instead of DMSO stock solutions. Handling steps for the solid materials are difficult to automate and typically become more labor-intensive and usually constrain throughput of thermodynamic solubility assays. Importantly, at this stage information on solubility becomes available for the first time with related information on solid state characteristics allowing solubility optimization strategies to commence [37, 38].
Assessment of solubility by the aforementioned kinetic or thermodynamic solubility assays is limited to generic conditions such as one pre-defined buffer system, typically at neutral pH. To get a physiologically more relevant understanding – especially for orally administered drugs – thermodynamic solubility is measured using bio-relevant conditions simulating the prevailing conditions within the gastrointestinal tract (GI-tract). Initially this is conducted using different buffers simulating the different pH conditions in the GI-tract. These buffers are typically simple inorganic or organic systems and they permit investigations of pH dependent solubility of the compound. The solubility of different salt forms can be initially assessed. As an example, for a weak base in the most acidic parts of the GI-tract (stomach), conversion of the parent form to the corresponding hydrochloride salt may take place. Accordingly, solubility as measured in the thermodynamic assay will refer to the salt form and not to the parent – and this information will be the physiologically relevant.
Application of Biopharmaceutical Solubility Approaches
As the GI-tract is a complicated biological system [39, 40], even the aforementioned approaches to determine pH-dependent solubility do not provide the complete picture of solubility behavior that will underpin drug absorption. Prediction of drug absorption, as a prerequisite to ensuring adequate bioavailability of a research compound, becomes critical during late stage preclinical research in combination with formulation development as part of pharmaceutical development. At this stage, further parameters influencing solubility need to be assessed. As a starting point, the influence of bile salts on drug solubility must be assessed. The best way to obtain this information would be using human GI-media in ex-vivo settings [41]. However, this approach is not practical during routine research and development. A much more practical way to investigate the impact of bile salts on solubility is provided by the using biorelevant dissolution media, which also can be easily utilized for thermodynamic solubility determinations. Biorelevant dissolution media such as FaSSIF (fasted state simulated intestinal fl uid), FeSSIF (fed state simulated intestinal fl uid), FaSGF (fasted state simulated gastric fl uid) and FeSGF (fed state simulated gastric fl uid) mimic the eff ect of bile salts, pH, ionic strength as well as osmolarity of human GI-fl uids [42]. When such biorelevant media have been introduced, preparation of these systems was often a laborious procedure. However, due to the availability of commercial ready-to-use kits, preparation of the media has been simplified and measurements of solubility in these media can be performed with throughput of 10-100 compounds per day [43]. For many compounds, a significant increase in solubility is seen due to the presence of bile salts. This especially holds true for lipophilic and basic moieties, where increases in solubility can be up to one or two orders of magnitude. Usage of bio-relevant media to determine thermodynamic solubility, allows the generation of physiologically relevant data, but also allows the initial assessment of potential food eff ects (FaSSIF vs. FeSSIF solubility).
At this stage of a project, further physicochemical characterization of research compounds are carried out for a very limited number of candidates and focus on intrinsic or apparent dissolution and the eff ect of particle size. Technical feasibility of these investigations has been facilitated during the last decade by the development of minidissolution apparatus and particle size determination using image analysis [44, 45], which provides important information using a few milligrams of the compound. These miniaturized approaches allow the assessment of both solubility and dissolution as part of preformulation activities, i.e. salt-selection, polymorphic form selection and the eff ect of particle size reduction.
Finally, methods to predict drug absorption and bioavailability have been improved significantly over the last decade. This includes approaches which go beyond allometric scaling [46] to the well known Biopharmaceutical Classification system (BSC) [47]. During the last few years, the BCS has been refined into the Development Classification System (DCS) [48], which addresses solubility, permeability and the dose of the drug. The DCS approach also takes into account dissolution rate and distinguishes between solubility and dissolution limited absorption. The use of pharmacokinetic simulation software has also become widespread [49] and might allow a more detailed understanding of the behavior of the research compound in humans and animals; including dissolution, solubility and an understanding of how a drug might precipitate in the GI-tract (due to shifts in physiological pH).
Conclusion
Solubility is one of the most important physicochemical parameters used across research and development. Historically, a large number of in-silico solubility methods have been employed. These computational methods provide comparative rank order assessments of solubility across different discovery programs and they need to be able to cope with significant numbers of compounds, with the objective of filteringout ‘non drug-like’ compounds. Although solubility is relatively easy to define, it can be difficult to predict using computational methods primarily due to solid state constraints (enthalpy and entropy considerations). The results from the recent Solubility Challenge demonstrate how difficult accurate solubility prediction can be and suggest that simpler in-silico methods may be more appropriate. In parallel, experimental methods to assess solubility have been improved and automated in recent years, and currently allow measurement of solubility for large numbers of compounds. This holds especially true for measurement of kinetic solubility. However, to ensure sufficient bioavailability of drugs, thermodynamic solubility becomes relevant. The use of kinetic and thermodynamic solubility should be clearly distinguished as the erroneous use of kinetic solubility for compound optimization can be misleading. For a deeper understanding of the role of solubility for drug absorption in animal and humans, methods and media to mimic in-vivo behavior have become more widespread and easy to use. Finally, drug absorption can be simulated and mechanistically understood based on solubility data and simulation software. Therefore simulation applications are widespread.
Author Biographies
David Elder, Ph.D., studied chemistry at Newcastle upon Tyne (BSc, MSc), before moving to Edinburgh to study for a Ph.D. in Crystallography. Dr. Elder has 35-years’ experience at a variety of pharmaceutical companies (Sterling, Syntex and GSK). He is currently a director within the Scinovo group in GSK R&D focused on externalization. He has seven patents to his name.
Christoph Saal, Ph.D., studied chemistry at the Technical University of Darmstadt and graduated in Physical Chemistry. In 1999, he joined Merck KGaA where he held several positions within Analytical and Medicinal Chemistry. Currently, Christoph Saal is heading a group focused on analytical and physicochemical characterization of New Chemical Entities.
References
- Lipinski C.A., Lombardo F., Dominy B.W., Feeney P.J. (1997). Experimental and computational approaches to estimate solubility and permeability in drug discovery and development settings. Adv. Drug Deliv. Rev. 23: 3–25.
- Edwards M.P., Price D.A. (2010). Role of Physicochemical Properties and Ligand Lipophilicity Efficiency in Addressing Drug Safety Risk. Ann. Rep. Med. Chem. 45: 381–391.
- Hopkins, A.L., Groom, C.R., Alex, A. (2004). Ligand efficiency: A useful metric for lead selection. Drug Discovery Today 9 (10): 430–431.
- Hill, A.P., Young, R.J. (2010). Getting physical in drug discovery: a contemporary perspective on solubility and hydrophobicity. Drug Discovery Today. 15: 648–655.
- Elder, D.P., Holm, R. (2013). Aqueous solubility: simple predictive methods (in silico, in vitro and bio-relevant approaches). Int. J. Pharm. 453: 3-11.
- Gleeson, M.P., Hersey, A., Montanari, D., Overington, J. (2011). Probing the links between in vitro potency, ADMET and physicochemical parameters. Nat. Rev. Drug Discov. 10: 197–208.
- Elder, D.P. (2010). Effective Formulation Development Strategies for Poorly Soluble Active Pharmaceutical Ingredients (APIs). American Pharmaceutical Review, 13 6(1): 28-34.
- Liu, R. (Editor) (2008). Water-insoluble drug formulation. CRC Press.
- Lamanna, C., Bellini, M., Padova, A., Westerberg, G., Maccari, L. (2008). Straightforward recursive partitioning model for discarding insoluble compounds in the drug discovery process. J. Med. Chem. 51, 2891–2897.
- Faller, B., Ertl, P. (2007). Computational approaches to determining drug solubility. Adv. Drug Deliv. Rev. 59, 533–545.
- Huuskonen, J. (2000). Estimation of aqueous solubility for a diverse set of organic compounds based on molecular topology. J. Chem. Inf. Comput. Sci. 40, 773–777.
- Klopman, G., Zhu, H. (2001). Estimation of aqueous solubility of organic compounds by the group contribution approach. J. Chem. Inf. Comput. Sci. 41, 439–445.
- Tetko, I.V., Tanchuk, V.Y., Kasheva, T.N., Villa, A.E.P. (2001). Estimation of aqueous solubility of chemical compounds using E-state indices. J. Chem. Inf. Comput. Sci. 41, 1488–1493.
- Lipinski C.A., Lombardo F., Dominy B.W., Feeney P.J. (2012). Experimental and computational approaches to estimate solubility and permeability in drug discovery and development settings. Adv. Drug Deliv. Rev. 64: 4-17.
- Llinàs, A., Glen, R.C., Goodman, J.M., 2008. Solubility challenge: can you predict solubilities of 32 molecules using a database of 100 reliable measurements? J. Chem. Inf. Model. 48, 1289–1303.
- Hopfinger, A.J., Esposito, E.X., Llinàs, A., Glen, R.C., Goodman, J.M. (2009). Findings of the challenge to predict aqueous solubility. J. Chem. Inf. Model. 49, 1–5.
- Hewitt, M., Cronin, M.T.D., Enoch, S.J., Madden, J.C., Roberts, D.W., Dearden, J.C. (2009). In silico prediction of aqueous solubility: the solubility challenge. J. Chem. Inf. Model. 49, 2572–2587.
- Hansch, C., Quinlan, J.E., Lawrence, G.L. (1968). Linear free energy relationship between partition coefficients and the aqueous solubility of organic liquids. J. Org. Chem. 33, 347–350.
- Cheng, T., Li, Q., Wang, Y., Bryant, S.H. (2011). Binary classification of aqueous solubility using support vector machines with reduction and recombinant feature selection. J. Chem. Inf. Model. 51, 229–236.
- Kramer, C., Beck, B., Clark, T. (2010). Insolubility classification with accurate prediction probabilities using a metaClassifier. J. Chem. Inf. Model. 50, 401–414.
- Hilfiker R. (Editor) (2006). Polymorphism in the pharmaceutical industry. Wiley-VCH.
- Chan H.C.S, Kendrick, J., Leusen, F.J.J. (2011). Predictability of the polymorphs of small organic compounds: Crystal structure predictions of four benchmark blind test molecules. Phys. Chem. Chem. Phys. 13, 20361-20370.
- Sanghvi, T., Jain, N., Yang, G., Yalkowski, S.H. (2003). Estimation of aqueous solubility by the General Solubility Equation (GSE) the easy way. QSAR Comb. Sci. 22, 258–262.
- Hill, A.P., Young, R.J. (2010). Getting physical in drug discovery: a contemporary perspective on solubility and hydrophobicity. Drug Discov. Today 15, 648–655.
- Leeson, P.D., Springthorpe, B. (2007). The influence of drug-like concepts on decision making in medicinal chemistry. Nat. Rev. Drug Discov. 6, 881–890.
- Ritchie, T.J., MacDonald, S.J.F. (2009). The impact of aromatic ring count on compound developability – are too many aromatic rings a liability in drug design? Drug Discov. Today 14, 1011–1020.
- Ritchie, T.J., MacDonald, S.J.F., Young, R.J., Pickett, S.D. (2011). The impact of aromatic ring count on compound developability: further insights by examining carbo- and heteroaromatic and -aliphatic ring types. Drug Discov. Today 16, 164–171.
- Lovering, F., Bikker, J., Humblet, C. (2009). Escape from the flatland: increasing saturation as an approach to improving clinical success. J. Med. Chem. 52, 6752–6756.
- Wassvik, C.M., Holmén, A.G., Draheim, R., Artursson, P., Bergström, C.A.S. (2008). Molecular characteristics for solid-state limited solubility. J. Med. Chem. 51, 3035–3039.
- Ishikawa M., Hashimoto, Y. (2011). Improvement in aqueous solubility in small molecule drug discovery programs by disruption of molecular planarity and symmetry. J. Med. Chem. 54, 1539-1554.
- Petereit A., Saal C. (2011). What ist he solubility of my compound? Assessing solubility for pharmaceutical research and development compounds. Am. Pharm. Rev. 14, 68-73.
- Hoellke B., Arlt M., Saal C. (2009). Comparison of nephelometric, UV-spectroscopic and HPLC methods for high-throughput determination of aqueous drug solubility in mictrotiter plates. Anal. Chem. 81, 3165-3172.
- Wan H., Holmen, A.G. (2009). High-throughput screening of physicochemical properties and in-vitro ADME profiling in drug discovery. Comb. Chem. High Through. Screen. 12, 315-329.
- Alsenz J., Kansy M. (2008). High-throughput solubility measurement in drug discovery and development. Adv. Drug Deliv. Rev. 59, 546-567.
- Pitt A. (2005). High-throughput screening to determine aqueous drug solubility. Pharm. Disc. 5, 46-49.
- Kerns E.H. (2001). High-throughput physicochemical profiling for drug discovery. J. Pharm. Sci. 90, 1838-1858.
- Petereit A., Saal C. (2012). Optimizing Solubility: Kinetic versus thermodynamic solubility – Temptations and risks. Eur. J. Pharm. Sci. 47, 589-595.
- Sugano K., Kato T., Suzuki K., Keiko K., Sujaku T., Mano T. (2006). High-throughput solubility measurement with automated polarized light microscopy analysis. J. Pharm. Sci. 95, 2114-2122.
- Diakidou M., Vertzoni M., Goumas K., Soederlind E., Abrahamsson B., Dressman J.B., Reppas C. (2009) Characterization of the contents of ascending colon to which drugs are exposed after oral administration to healthy adults. Pharm. Res. 26, 2141-2151.
- Kalantzi L., Goumas K., Kalioras, V., Abrahamsson B., Dressman J.B., Reppas C. (2006) Characterization of the human upper gastrointestinal contents under conditions simulating bioavailability/bioequivalence studies. Pharm. Res. 23, 165-176.
- Dimitrios P., Vertzoni, M., Goumas K., Kalioras V., Beato S., Buttler J. Reppas C. (2011) Precipitation in and supersaturation of contents of the upper small intestine after administration of two weak bases to fasted adults. Pharm. Res. 28, 3145-3158.
- Jantratid E., Janssen N., Reppas C., Dressman, J.B. (2008) Dissolution media simulating conditions in the proximal human gastrointestinal tract: an update. Pharm. Res. 25, 1663-1676.
- Ottaviani G., Gosling D.J., Pattisier C., Rodde S., Zhou L., Faller B. (2010) What is modulating solubility in simulated intestinal fluids? Eur. J. Pharm. Sci. 41, 452-457.
- Avdeef A., Tsinman K., Tsinman O., Sun N., Voloboy D. (2009) Miniaturization of powder dissolution measurement and estimation of particle size. Chemistry & Biodiversity 6, 1796-1811.
- Becker A., Petereit. A, Saal C. Gaining knowledge on drug absorption: Gathering physicochemical data for PK simulations. Am. Pharm. Rev. 15, 1194-1198.
- West G.B., Brown J.H., Enquist B.J. (1997) A general model for the origin of allometric scaling laws in biology. Science 276, 122-126.
- Amidon G.L., Lennernas H., Shah V.P., Crison J.R. (1995) A theoretical basis for a biopharmaceutical drug classification: The correlation of in-vitro drug product dissolution and in-vivo bioavailability. Pharm. Res. 12, 413-420.
- Buttler J.M., Dressman J.B. (2010) The developability classification system: Application of biopharmaceutical concepts to formulation development. J. Pharm. Sci. 99, 4940-4954.
- Parrott N., Lave T., (2010) Computer models for predicting drug absorption. Drugs Pharm. Sci. 193, 338-355